“Modern MMM (Marketing Mix Modeling)” Practical Guide for Marketers
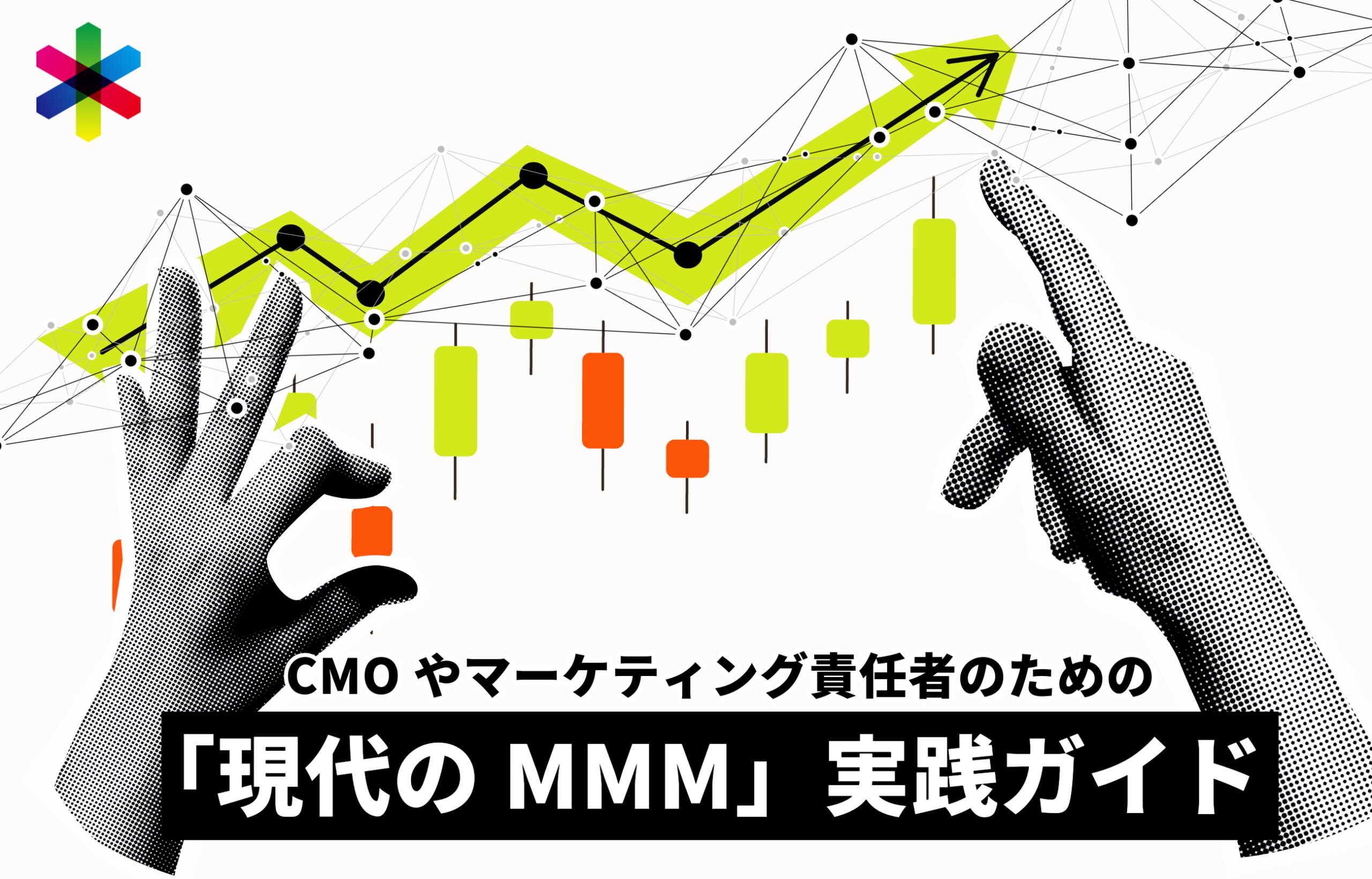
Introducing a "practical guide" for CMOs and marketing managers who want to utilize MMM (Marketing Mix Modeling) to optimize marketing strategies and improve business results.
In recent years, many companies have focused on MMM, and this is largely influenced by two factors. The first is the increasing complexity of consumer consumption behavior and the diversification of marketing channels. As the points of contact between companies/brands and consumers become more diverse, MMM, which can measure the effectiveness of each marketing measure, including indirect effects, has become a useful method for optimizing investments. And second, recent privacy changes like GDPR and the Cookie Regulation. Leveraging MMM is even more important for today's businesses, which must embrace ways to reduce their reliance on individual tracking data.
Since its founding in 2012, XICA has been providing solutions specialized in the data science field, and has supported more than 250 major corporate clients based on its proprietary MMM. Ta. This practical guide is not limited to XICA's services, but has been compiled as a guide for optimally utilizing the MMM method. We hope that this practical guide will provide guidance for those who are introducing MMM in one of these ways, or for those who have already had experience in using MMM to achieve results.
This articleClick here if you would like to download the document version.
table of contents
- 1 Introduction to MMM (Marketing Mix Modeling)
- 2 Clarify the purpose of the MMM (Marketing Mix Modeling) project
- 3 Current status of MMM (Marketing Mix Modeling)
- 4 Collect the right data
- 5 Collaboration with internal teams and MMM (marketing mix modeling) providers
- 6 Incorporate MMM (Marketing Mix Modeling) into the decision-making process
- 7 Utilize external experts in MMM (Marketing Mix Modeling)
- 8 Summary
1 Introduction to MMM (Marketing Mix Modeling)
1.1 MMM past and present
MMM is a statistical method used to quantify the impact of various marketing measures on sales, market share, and other business KPIs/KGIs. MMM identifies which marketing measures are most effective, makes data-based decisions in allocating marketing budgets, and optimizes the marketing mix. Additionally, MMM can predict the impact of future marketing initiatives and sales, aiding in budgeting and long-term planning.
The history of MMM dates back to the 1950s. In its early days, MMM focused on measuring the impact of marketing initiatives such as TV commercials and print ads for food, beverage, and household goods brands using linear regression and econometric techniques. Modern MMMs have evolved over time to incorporate more diverse data sources, automated analysis, and more sophisticated statistical techniques. In 2016, XICA launched the MMM solution “MAGELLAN” that utilized proprietary computer science, and in recent years, in 2021.Meta Robyn open source libraryIn 2022, Google will publish Bayesian MMM papers andLightweightMMM open source libraryMMM solutions have been further developed in recent years, including the release of .
1.2 Advantages and disadvantages of MMM
Since the 1950s, MMM has been used to optimize the marketing mix and maximize cost effectiveness, but what other benefits does it offer? Also, why has MMM not been widely adopted in Japan? Here we will explain the advantages and disadvantages of using MMM to help you decide whether it is right for your company.
The biggest advantage of MMM is that it allows for the overall optimization of marketing investments. Since MMM can evaluate all marketing channels side by side, it is possible to derive the optimal budget allocation between each channel (including channels such as TV and OOH, where effectiveness is difficult to measure) and measures. In many cases, the methods of measuring effectiveness, the metrics measured, and the content of reports vary depending on the advertising platform or channel. As a result, individual optimization for each channel progresses, making it difficult to prioritize channels with overall optimization in mind. Companies with complex marketing mixes rely on MMM as a source of information to identify priorities across channels.
MMM also has the advantage of being able to calculate the necessary marketing budget based on scientific evidence. Many companies set marketing budgets as a percentage of sales targets. For example, if a company allocates 10% of sales to marketing budget, and the company is planning sales of 100 billion yen, the marketing budget would be set at 10 billion yen, which is 10% of sales. With this type of budget allocation, there is no way to know whether you could have achieved your goals with less money, or how much of that budget would have been appropriate. By using MMM, you will be able to make scientific budget decisions by predicting how much your marketing will contribute to sales.
However, MMM is not a panacea and has its drawbacks. For example, since MMM analyzes based on past data, it is not possible to predict the results of measures that have not been implemented in the past. In addition, it is necessary to collect high-quality data for analysis. Gathering the data required for MMM can be time-consuming, especially for companies without a centralized data management or data warehouse, as they need to collaborate with various departments and teams. . In addition, you may need to collect data from external sources on external factors such as macroeconomic indicators, market and industry trends, holidays and seasonal factors, and weather. You may also need to purchase data about your competitors' practices. This data collection process is the most time-consuming part when implementing MMM for the first time.
Additionally, MMM may not be suitable for companies with little data or less complex marketing, such as companies with monthly marketing investments of less than 1,000 million yen or companies with three or fewer advertising channels.
Furthermore, MMM analysis may reflect internal biases in model construction and variable selection. A large part of the changes in the modernization of MMM have been to remove or reduce the impact of these human biases by automating much of the variable selection and modeling process. Another way to avoid bias is to use trusted external experts.
MMM is still in the process of modernizing, and not all companies have adopted best practices. Even companies implementing cutting-edge MMM are still wondering how best to explain the impact of advertising creatives, how to consider the long-term effects of branding advertising, and how to optimize it. Discussions remain, such as how to obtain more detailed policy data. In recent years, big tech companies like Meta and Google have been investing in this space, as well as data science consulting companies like XICA. Innovation and improvement in MMM methods will continue to accelerate.
1.3 Why MMM is difficult
MMM is an effort to statistically measure the relationship between marketing activities and purchasing behavior. As mentioned above, modern marketing structures have become extremely complex due to the diversification of contact points between companies and brands and consumers. Therefore, it is also complicated to express it statistically. Some people think that advances in machine learning can be applied to this problem, but unfortunately it's not that easy. Most machine learning tools only make predictions and cannot explain the "why" behind the analyzed data or results. In order to explain this ``why,'' knowledge of marketing and business and assumptions based on that knowledge are important. In other words, in order to accurately measure the effectiveness of marketing activities using MMM, and to interpret and explain the results, you need not only an understanding of specialized algorithms, but also knowledge about your company's business and marketing.
There are also difficulties in building models to conduct analyzes that match the actual situation. For example, short-term and Model construction becomes more difficult in order to consider and analyze more than just direct effects.
Additionally, MMM analysis must also consider external factors that affect sales, such as seasonality, competitor activity, and external events. Analyzing without taking these factors into account may lead to incorrect conclusions, such as overestimating or underestimating the marketing measures taken.
With these points in mind, consider how you can implement MMM in your company. For the purpose of analysis, it is important to formulate hypotheses in advance, such as what elements should be included in the model and what variables are considered to have a large influence. When thinking about this, please refer to the following practical guide as a guide.
2 Clarify the purpose of the MMM (Marketing Mix Modeling) project
2.1 Define what you want to achieve with MMM
MMM is a very important initiative for companies aiming to maximize ROI (return on investment).
As with data analysis in general, clearly defining your objectives helps align your efforts with your business goals. Also, if the purpose is clear, what kind of data should be collected, what indicators should be tracked, how should the analysis results be interpreted, and most importantly, what actions should be taken? It also serves as a guideline from this perspective. When setting objectives, it's important to consider the long-term and short-term goals of your business. For example, a short-term goal might be customer acquisition, while a long-term goal might be sales growth or brand awareness. Avoid optimizing only for short-term goals and ignoring the medium- to long-term impact of your marketing.
One of the most common mistakes in MMM is building a model that tries to answer every question, only to end up being unable to effectively answer any of the questions. When using MMM, it is important to focus on the most important and actionable questions for your business. You might ask yourself, "If we knew the answer to this question, what action would we take?" For example, it may be interesting to know how much inflation affected sales in the previous period, but if that information is not useful for action in the current or next period, it is probably not an important question for the business. is.
Once you have determined your purpose, you can consider things like what variables should be included in the model, what scenarios should be simulated, and what output (charts, graphs, etc.) should be used to explain it. This makes it easier to utilize the analysis results in decision making. Conversely, if you do not define the purpose before starting analysis with MMM, you run the risk of ending up with analysis results that cannot be used for final decision-making. Such failures reduce the reliability of the MMM analysis method itself, and may also result in the loss of future growth opportunities using MMM.
2.2 Identify the most important metrics (KPIs) for your efforts
To create a practical model, you must first identify the most important performance indicators (KPIs) for your company. MMM models the relationship between KPI, the objective variable, and marketing activities, the explanatory variable.
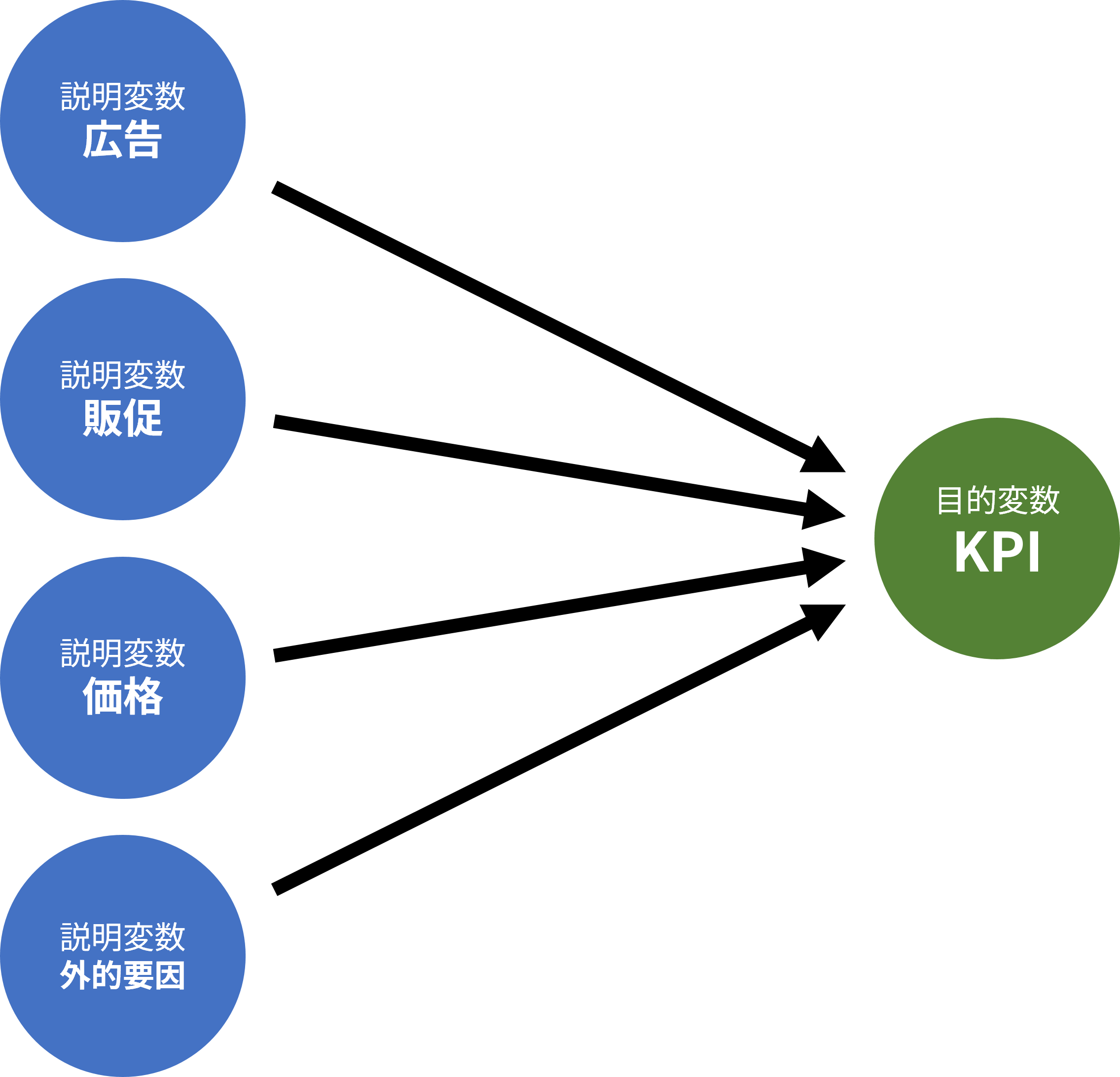
When deciding on KPIs, it is generally best to align them with indicators of business goals. Sales and number of units sold are often set, but in the case of a business that takes a long time (several months to several years) to lead to sales, it is recommended to use an alternative indicator that correlates to sales, which is the business goal, as a KPI. is recommended. For example, in the case of a B2B business, after acquiring potential customers through marketing, it can take months or even years for them to actually lead to sales. Additionally, in B2C subscription businesses, the timing of payment (increase in sales) differs depending on the customer using the service, so it may be difficult to use sales during the analysis period as a KPI. In such cases, it is appropriate to set alternative metrics such as "qualified leads (e.g. MQL)" or "active users (e.g. MAU)" as objective variables for MMM.
Additionally, KPIs may vary depending on the company's phase and marketing objectives. For example, if you have a fast-growing e-commerce business, you are likely focusing on acquiring new customers, so you might want to use first order or first average unit price as KPIs. On the other hand, even if the company is in the same e-commerce business, if the business is mature, it would be better to focus mainly on repeat purchases and use repeat orders and average unit price as KPIs.
Identifying the most important KPIs for a company is a crucial step in operating an MMM. By setting the optimal KPI for your business, you will be able to utilize the results of MMM analysis to optimize your marketing.
2.3 Estimate the resources to allocate to the MMM project
MMMs vary in size and complexity depending on the company and business. Allocating the right resources is critical to project success. There are several points to consider when calculating the required resources.
The first isSize and complexity of marketing efforts.
As the scale of your marketing efforts grows, you will need to consider numerous advertising channels, marketing strategies, and models that take into account various factors. Additionally, the more complex the marketing activity, the more likely the MMM will face challenges with multicollinearity (too many variables being correlated with each other), and the more time and resources it will require to verify them. Masu. Additionally, if you have a large marketing budget, a 1% error in model accuracy can cost tens of millions to hundreds of millions of yen, so it is important to maintain high model accuracy.
On the other hand, if the scale of your marketing efforts is not large, investing in MMM may not provide significant benefits. If you only advertise on a few channels, you may not need MMM to analyze effectiveness and optimize your investments. Also, related to the second point mentioned below, when the scale of marketing activities is small, the available data is often limited, in which case it is not possible to build a sufficient model and it is difficult to utilize the analysis results. You may find yourself unable to do so. In such cases, first of all, it is relatively resource-intensive.Multiple regression analysis in ExcelIt is also effective to utilize and measure the effects.
The second point isQuantity and quality of data available for analysis will be important.
For an MMM project to be successful, it is important to use sufficient quantity and quality data. A typical MMM requires at least one year's worth of daily or weekly historical data for each marketing channel. If your data is limited, you may need to devote more resources to data collection and cleansing (identifying and correcting corrupt or inaccurate data). Depending on data management, data collection can take anywhere from a few weeks to a month.
The third point isStatistical expertise required for MMM projects will be important.
In-house expertise in statistics, econometrics, and data science may allow you to allocate fewer external resources to your project. However, if you lack expertise in these areas, you may need to hire outside consultants or devote resources to training your employees.
After considering these points, the final important thing isEstimating the results of MMM analysis (profit improvement through marketing optimization)It is that.
Let's take the example of a company that spends 10 billion yen per year on marketing budget and has annual sales of 50 billion yen (ROAS: 500%). If a company with a ROAS of 500% uses 1,000 million yen of its marketing budget for an MMM project, the sales would be 5,000 million yen (1,000 million yen x 500%) if the same amount of money had been spent on promotions. will be deleted. In other words, in order to justify the cost of an MMM project, marketing results must improve by more than 6,000 million yen (1,000 million yen + 5,000 million yen) (1.2% or more) in sales. If you think about it the other way around, for example, if sales improve by 2% by optimizing marketing investment, the cost of the MMM project will be fully profitable, and if it is expected to improve by 5% or 10%, then proactively implement it. The decision is that it should be done. Of course, it is not possible to accurately estimate the amount of improvement before conducting an analysis, but estimating the amount of improvement you should aim for is important in determining whether or not to implement and continue the project. For reference, the average sales improvement suggested by MMM analysis in XICA's support of more than 250 companies is 4-8%.
3 Current status of MMM (Marketing Mix Modeling)
3.1 From econometrics to modern MMM
From here on, we will delve deeper into the opening topic, "#1.1 MMM's past and present."
The MMM approach isIt evolved from econometrics.. Econometrics is a set of statistical methods based on linear regression analysis, and is applied not only to marketing but also to a variety of fields such as finance, agriculture, and medicine. One application to marketing is MMM.
To this day, the fundamental method has remained largely unchanged. What has changed is the infrastructure that supports the field. Traditional econometric models require highly trained econometricians and statisticians to manually build the model and adjust all data inputs and variables. This made it an expensive and time-consuming method, and it was common for analysis to be performed only once a year. Nowadays, in a marketing environment that is rapidly changing due to digitalization, there is a need to increase the pace of this analysis, and as a result, solutions have been created that systemize MMM and automate analysis. By systematizing the modeling process, these solutions have significantly reduced the cost of building models.
In recent years, open source MMM libraries such as Meta's Robyn and Google's LightweigthMMM have become available, and anyone with knowledge of statistics or programming languages can now run MMM for free and speedily. Ta.
Additionally, data collection and cleansing tasks, which used to take time, are becoming easier with advances in digital transformation (DX). MMM can now be updated more frequently by automating the process of collecting and integrating data from multiple sources.
3.2 Checkpoints of modern MMM functionality
Here, we will introduce checkpoints when selecting an MMM solution. Please note that if you run without taking these checkpoints into account, there is a risk that the analysis results will be useless or lead to incorrect results.
By knowing the points introduced here, you will be able to make more appropriate decisions and instructions when selecting an MMM solution or coordinating with your data science team. We will introduce the points to consider, divided into "model analysis function" and "output function".
Model analysis features
- Are the ripple effects (indirect effects) of marketing measures taken into account?
In particular, upper funnel measures such as awareness measures not only have a direct effect on sales, but also have indirect effects such as boosting the effectiveness of digital advertising and the number of designated searches. If indirect effects are not taken into account in MMM analysis, the effects of reaping measures such as designated search advertising and in-store sales promotion measures may be overestimated. - Are the residual effects of marketing measures (ad stock, carryover) taken into account?
The effectiveness of marketing initiatives remains, albeit attenuated, for days to weeks (or even months) after contact with consumers. Unless the analysis takes into account the residual effectiveness of marketing strategies, such as people remembering brands or products they saw advertised in the past while shopping in stores or online, it may not be possible to evaluate the effectiveness of marketing strategies. These residual effects vary depending on the publishing medium, creative, industry, product category, etc. - Does it take into account saturation (non-linear relationships, diminishing returns)?
It goes without saying, but just because you spend 10 times more on marketing initiatives doesn't necessarily mean you'll get 10 times more results. The more you increase the amount invested, the more the results will reach a plateau once a certain level is exceeded due to the law of diminishing returns, and the ROI will worsen. If such a non-linear relationship between cost and effectiveness is taken into account in MMM, sales forecasts based on budget allocation optimization can be simulated with higher accuracy. On the other hand, if you use analysis results that have not taken saturation into account, you may end up over-investing in measures that are not expected to be any more effective. - Is brand equity (brand accumulation effect) taken into account?
Awareness and favorability determined through questionnaire surveys are often used as indicators to measure brand power. However, it is difficult to explain how brand power leads to business results using these indicators alone. One way to explain this is to measure the cumulative effect of branding, in which continuous investment in branding measures leads to an increase in a company's baseline sales over the medium to long term, ranging from several months to several years. By taking these effects into account and analyzing them, you will be able to explain the contribution of branding initiatives to your business and their cost effectiveness when drawing up medium- to long-term marketing strategies.
*The brand equity analysis function described here is a function of XICA's MMM solution. Other MMM solutions may incorporate factors such as number of named searches or periodic awareness surveys into their models as proxy indicators of brand effectiveness.
- Can seasonality be taken into account?
MMM requires consideration of the relationship between marketing and seasonality. In general, you'll tend to spend more of your budget when consumers are most likely to buy your product (for example, promote ice cream in the summer and sweaters in the winter). However, directly assessing the impact of seasonality on business results using MMM is likely to result in marketing being less cost-effective during periods of high demand. This is because most MMMs analyze the effect of seasonality as an independent variable without considering its impact on marketing measures. If you have a hypothesis that seasonality affects marketing, we recommend choosing an MMM that can take seasonal effects into account. - How often is the model updated?
In order to translate MMM analysis results into action, it is often no longer sufficient to update the model once a year, as was the case in the past. Especially now that the proportion of digital marketing is increasing, it is now possible to make decisions regarding budget allocation on a monthly, weekly, or even daily basis, making it possible to analyze the effectiveness of measures through MMM. Ideally, it can be done as quickly as possible.
Model output function
- Is there an error rate that indicates the accuracy of the model?
Confirming the accuracy of the model is very important when using MMM. Let's check the error rate and confidence interval of the estimated value that comes out as a result of the analysis. In general, the ROI estimates calculated from the model will be more accurate for measures that have a large cost or a large amount of data, and will be less accurate for measures that have a small cost and a small amount of data. By checking the accuracy of the analysis results, you can decide what and how much of the information you should refer to, and make appropriate decisions. - Is it possible to optimize sales forecasts and budget allocation?
MMM can predict how much results will be produced by marketing measures. This is extremely useful for budgeting and planning, as well as for reviewing policies based on progress during the period.
Furthermore, MMM is extremely useful because it allows calculation of optimal budget allocation under constraints that reflect realistic circumstances. For example, there are often constraints such as ``I want to adjust (suppress) the TV commercial budget by taking saturation into consideration, but the quarterly TV commercial budget has already been determined.'' Even when there are such constraints, MMM is useful because it can realistically optimize budget allocation based on the constraints.
3.3 MMM Project Timeline and Main Milestones
Successful MMM projects require advance planning and execution management.
Below are the major milestones and timelines for the MMM project. The timeline is an example of a project using a systemized MMM, but it is likely to be longer for a project manually conducted by a traditional consultant.
STEP 1: Set project goals and determine analysis scope (1 to 2 weeks)
The first step in any MMM project is to define the purpose of the effort and the key hypotheses to be tested. This includes understanding the company's business goals, sorting out the problems and issues associated with them, designing a model after understanding whether data can be obtained, and determining the scope of analysis and report content. This step usually takes 1-2 weeks.
STEP 2: Data collection and cleansing (2-4 weeks)
Data collection is an integral part of the MMM project. At least one year's worth of time-series data on results (sales, etc.), marketing and advertising measures, and external factors such as macroeconomics must be obtained. It is also important to cleanse the data you collect, as inaccurate data (missing values, typos, inconsistencies and duplications, etc.) can lead to inaccurate analysis results. This step usually takes 1-2 weeks.
STEP 3: Model construction and model confirmation (4 to 6 weeks)
Once you have collected and cleansed your data, you can begin your modeling work. This is an iterative process where the model is constantly re-run, tweaked and improved. In some cases, you may need to acquire new data on important variables that were omitted from your model. This step typically takes 4-6 weeks, depending on the scope of the analysis and the complexity of the model.
STEP 4: Analysis, report and suggestion (1-2 weeks)
The next step is to analyze the model's data to generate actionable recommendations based on your project goals and hypotheses. There are a variety of indicators and outputs that can be analyzed depending on the project goal and the hypothesis you want to test. It usually takes one to two weeks to create a report with analysis results and suggestions.
4 Collect the right data
4.1 Type and amount of data required for MMM
The main data required for MMM is marketing data, performance data, and data on external factors.
marketing dataincludes data about marketing activities such as advertising, promotions, and sales prices. This data can be collected from a variety of sources, including internal marketing reports, advertising agencies, third-party data providers, and advertising and social media platforms.
Results dataIncludes metrics such as sales, units sold, and customer acquisitions. This data can be collected from sources such as internal sales reports, POS systems, and CRM systems.
External factor dataincludes data about consumer trends, competitor activity, and other factors that can affect your marketing effectiveness and business results, such as holidays and weather.
To make your data collection process more efficient, you may want to consider setting up a data warehouse. This way, all the data is collected in one place in the appropriate format and can then be used for relatively labor-intensive modeling.
In order to build a reliable statistical model, it is said that at least 12 months of data is sufficient, but to identify seasonal patterns with higher accuracy, two or more years is recommended. Masu. As a general rule, the more data you have, the more accurately you can estimate the impact of marketing on business results.
4.2 Cleanse data collected from various sources
Cleansing data collected from various sources is essential to building reliable statistical models.
As mentioned above, the data you incorporate into MMM can come from a variety of sources, including internal databases, third-party data providers, advertising platforms, and agencies. Although it takes a considerable amount of effort to collect it, it is important to ensure the accuracy, completeness, and consistency of the data in any modeling effort. This includes removing duplicate data, correcting errors, standardizing data formats from different sources, etc. Cleansing and preparing data for inclusion in MMM is a time-consuming and resource-intensive task, so sufficient resources must be allocated to this task. At XICA, we have a team of dedicated data analysts and data scientists who support the cleansing and preparation of data received from our clients.
Below are a series of steps for data cleansing and preparation that can also be applied to MMM and other data analysis.
STEP 1: Inspect the data
The first step is to inspect your data and gain a rough understanding of their structure, format, and content. This includes looking for outliers such as missing data, outliers, and errors.
STEP 2: Standardization of data format
The next step is to get your data in a consistent format so you can analyze it. This includes standardizing column names, converting formats, removing duplicates, etc.
STEP 3: Convert data
Transform the data to meet the requirements of your analytical method. This includes aggregating data for multiple variables, creating new variables, and more.
STEP 4: Fill in missing values
Impute missing data items with estimates that are as realistic as possible based on the data you have.
STEP 5: Data verification
Re-examine the data to ensure it meets data standards for analysis.
STEP 6: Export data
Export cleansed data in a format that is easy to use for analysis, such as a CSV file or data warehouse.
4.3 Consider external data such as competitors and consumer trends
MMM focuses on the use of marketing and outcome data, but here we will explain the use of data related to external factors.
For example, a company in the tourism industry may want to incorporate "exchange rate fluctuations", which have a significant impact on the behavior of overseas consumers, into MMM. Similarly, for businesses with strong seasonality, as a proxy indicator of the seasonality of the industry,Google trendsYou can also incorporate search data into MMM. For example, companies in the automobile industry tend to see an increase in searches for "purchasing a car" from January to March every year, and by analyzing this trend while taking into account external factors, it is possible to accurately assess the effectiveness of measures. can be evaluated.
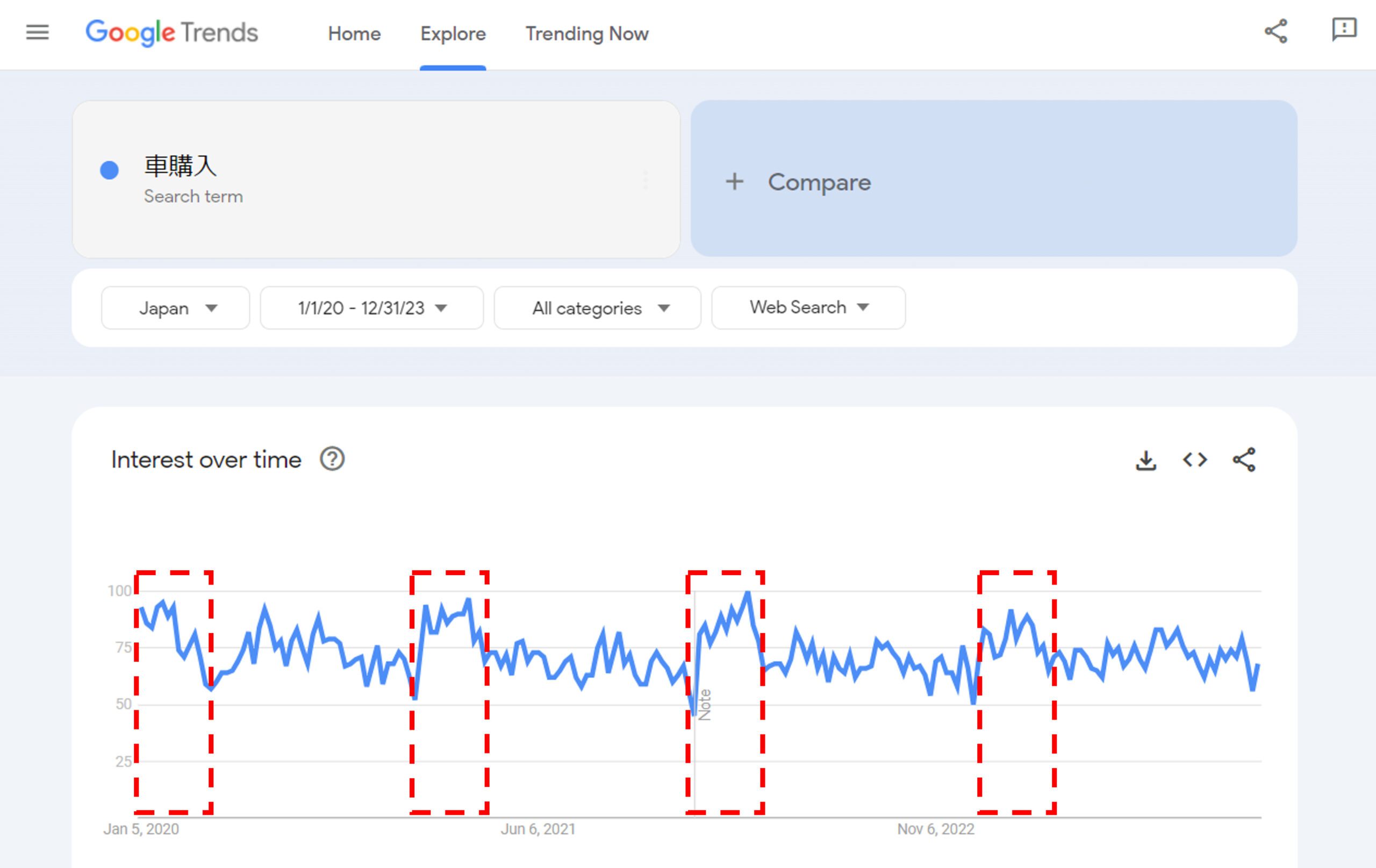
Competitor, consumer trends, and economic data can also provide valuable insight into the effectiveness of marketing efforts. For example, if a competitor runs a large amount of TV commercials or carries out large-scale promotions, this may have a negative impact on sales by more than the contribution of your own marketing activities. Consumer trend data, such as economic data such as unemployment rate, GDP growth rate, and inflation rate, as well as target customer preferences and behavior, can affect the effectiveness of marketing measures and sales, so it is important to incorporate them into MMM. Sometimes it's good.
note:Please keep in mind that analyzing more factors with MMM is not necessarily better. Because complexity and potential noise increase with increasing data sources, the models we build with our clients are careful to include only those variables that we believe will have an impact on the hypothesis we are testing.
5 Collaboration with internal teams and MMM (marketing mix modeling) providers
5.1 Collaboration with MMM project stakeholders
MMM analysis results are often explained and reported not only to the marketing department but also to a wide range of stakeholders within the company, including management and the accounting department. In order to translate the analysis results into action while moving the company, it is essential that these stakeholders understand the project. When proceeding with an MMM project, it is important to explain the purpose of the project and its effects on business issues to the relevant parties in advance and gain their understanding. In some cases, it may be a good idea to have stakeholders attend regular meetings or meetings to finalize the model. It is expected that the confidence of those involved in the model and analysis content will increase, and the degree of satisfaction with the results will increase.
With XICA's MMM solution, a dedicated project team (including consultants, data analysts, and data scientists) for each project conducts briefings and reporting sessions not only for the client's department in charge but also for each stakeholder. We support the creation of common understanding within our clients' companies.
5.2 Report analysis results to stakeholders
Once the MMM analysis is complete, it is important to report the results to stakeholders and gain their understanding. Present the findings from your analysis, the suggestions obtained, and the actionable actions that will lead to business results.
Choosing appropriate charts and graphs is important to clearly convey the key implications of the model. Bar graphs and waterfall charts are suitable for showing the contribution of each marketing channel to sales. Line graphs are also suitable for showing changes in performance over time.
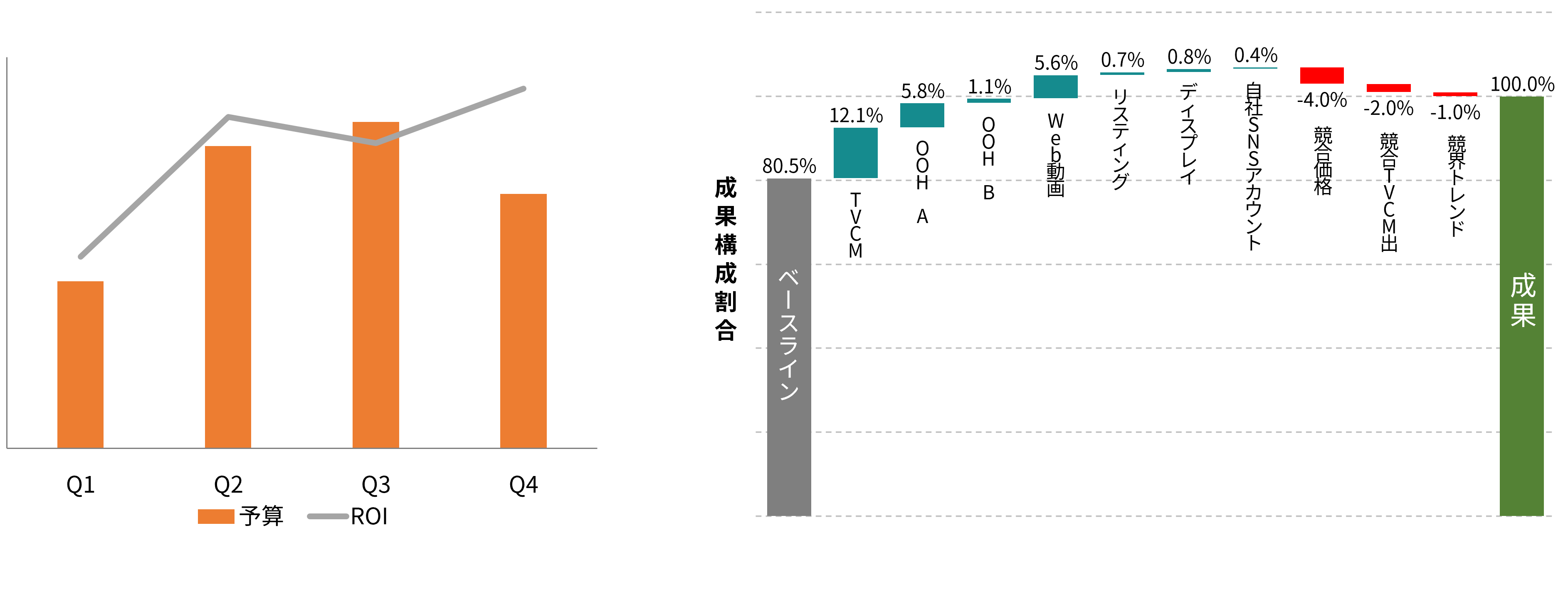
5.3 Respond to questions and comments regarding analysis results
Even if you involve stakeholders from the beginning, MMM projects may encounter objections or skepticism from other departments. This type of opinion is particularly likely to occur when the results of the analysis or suggestions obtained are unexpected or difficult to understand. It is important to be prepared to provide evidence-based explanations in response to questions and opinions from stakeholders.
If you anticipate that there will be skepticism in advance, it is also effective to have them participate in the project from the model construction stage, as described above. This will help you gain confidence in your models and analysis methods, and make it easier to accept your analysis results.
Also, if you lack information for decision-making, consider other alternative analyzes and share how you would respond.
6 Incorporate MMM (Marketing Mix Modeling) into the decision-making process
6.1 Establish a mechanism for regularly updating the model
In traditional econometrics, building models requires enormous costs and specialized knowledge, so models are updated only once a year, or at least once every six months. In modern marketing, where the environment changes rapidly, analysis results cannot be used for action with this update frequency. To fully utilize the benefits of MMM, it is important to keep the model aligned with the latest business conditions. To do this, it is necessary to have a system in place to update the model regularly.
First, determine the frequency of data updates. For example, if you're in a rapidly changing market, you might consider updating your model monthly or weekly. On the other hand, if you are in a relatively stable market, quarterly or semi-annual updates may be sufficient.
You should also consider the complexity of your marketing efforts. Updating a model is relatively easy if it is simple, easy to understand, and changes little. On the other hand, if your model is complex and new channels are frequently introduced, you may need to work with in-house data scientists or an external MMM provider to maintain an optimal model.
6.2 Use for marketing strategy formulation and budget allocation optimization
By understanding the factors that lead to business results through MMM analysis, you will be able to make optimal data-based decisions regarding marketing strategy formulation and budget allocation.
Using MMM, you can understand the impact of different marketing initiatives, creatives, and messages on business results. For example, you can predict the impact of various marketing scenarios, such as how much sales will be affected if you suppress advertising. This information can help you decide which initiatives to invest in and how to communicate.
note:It's important to keep in mind that MMM is an iterative endeavor. When making decisions about marketing strategy and budget allocation, it's essential to update your models regularly to reflect the latest data and business conditions.
6.3 Tune the model to improve reliability
Increasing confidence in model analysis results is especially important when the company is at high risk or requires a large financial decision. Confidence can be increased by checking the model's predictive accuracy through backtesting, validating the model's results through lift surveys, area distribution tests, etc., and confirming the accuracy of recommended actions.
Backtesting is a method of evaluating the accuracy of a model using past data. The model is trained on data from a certain past point in time (for example, one month ago), and then generates predictions for one month ahead. By comparing the predicted values output by the model with the actually measured results, you can evaluate the predictive accuracy of the model. This is a method often used in the financial industry and trading to verify the accuracy and effectiveness of predictive models.
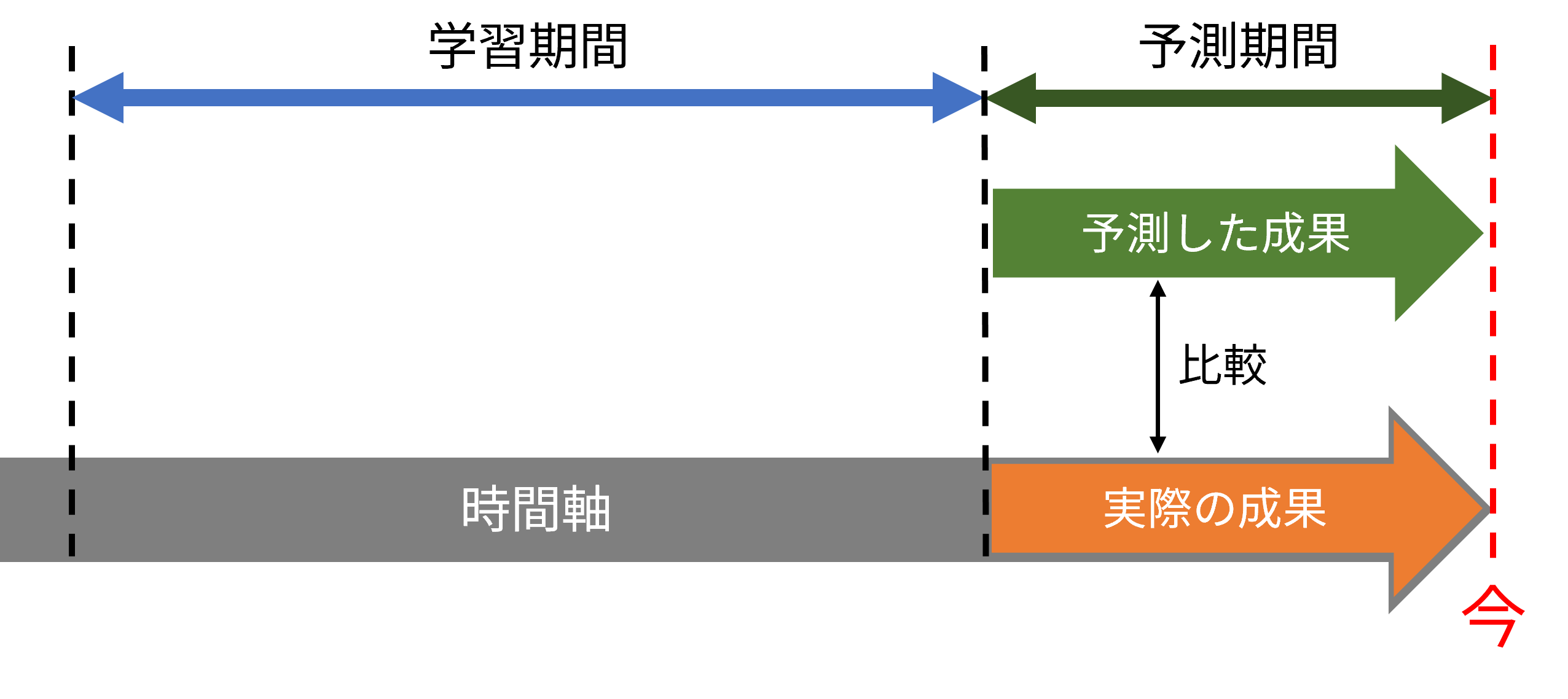
Lift studies compare the effects of a test group exposed to a specific marketing initiative to a control group that was not exposed. This allows you to measure the incremental impact of your measures on KPIs, compare it to the model's analysis results, and determine whether the recommended actions are accurate.
Area distribution testing involves testing a model's recommended actions in a specific area (such as a country or region) and comparing the results to other areas that have not implemented the action. This allows you to verify the accuracy of the model's recommended actions.
By adjusting your model through these tests, you can build a more reliable model and confidently take action.
note:It is important to keep in mind that any effectiveness analysis method, including the methods mentioned above, is not necessarily perfect. What you need to be careful about is when the results of MMM analysis are significantly different from those of other effectiveness analysis methods or the marketer's intuition. In these cases, it is important to investigate and decide whether to improve the model or consider the results as important findings.
6.4 Making MMM analysis results easily available to marketers
An effective way to incorporate MMM into the decision-making process is to make its analysis easily available to marketers.
For example, by building a dashboard with data visualization functions and budget and sales forecast simulation functions, marketers can freely check the effectiveness of past marketing activities without having technical knowledge. , you will be able to formulate hypotheses and simulate future investment scenarios.
Building such a dashboard is considered an effective way to utilize MMM in the decision-making process.
7 Utilize external experts in MMM (Marketing Mix Modeling)
7.1 Decide whether it is more reasonable to do it in-house or outsource it
When introducing MMM, consider whether to build it in-house or outsource it. As mentioned above, MMM projects vary in size and complexity, so it is difficult to say which one is better than the other. Consider factors such as the expertise and resources available in-house and the complexity of your marketing mix.
If your company's marketing mix is relatively simple and easy to understand, it may be easy to build a model within your company. On the other hand, if the marketing mix is complex and needs to include many variables, it may be better to ask or outsource the opinion of an outside expert.
7.2 Consult with experts and consulting firms to improve model accuracy and reliability
Even if you have the necessary resources in-house, if you want to improve the accuracy and reliability of your model, it is effective to seek guidance from external experts such as consulting companies who have a third-party perspective and expertise. In particular, the larger the investment in marketing, the more important a third-party perspective and expertise become.
When seeking external expertise, it is important to carefully evaluate the experience and reliability of the MMM solution provider. This includes a track record of successfully delivering MMM projects and expertise in your industry or business area.
7.3 Select an outsourced MMM solution
If you decide to seek outside help to build your MMM, it's important to choose a solution provider that fits your needs.
First, check whether the price and fee structure (monthly fee, fee per model update, fee per project, etc.) is suitable for your company. For information on considering how much resources and costs should be allocated, seeEstimate the resources to allocate to the MMM project” Let's check it again.
And the functionality of the MMM solution is also an important consideration. Different solution providers have different features, such as advanced modeling capabilities, simulation capabilities, frequency of reporting, and provision of proprietary data. Check whether the features provided match your needs and requirements.
The quality of customer support is also an important point to consider. Evaluation points include consulting in the analysis area, model customization, interpretation of analysis results, and suggestions.
Furthermore, it is important to consider the neutrality (third-party standpoint) of the outsourcing company. If there is a relationship that could create a conflict of interest (such as contracting out an executive function such as advertising), this could introduce bias in model construction and interpretation of results. It is important to select a subcontractor based on the relationship with your company.
8 Summary
MMM has attracted increasing attention in recent years as a method for evaluating the effectiveness of privacy-friendly marketing activities.
There are various ways to implement MMM, from building models in-house to operating using open source tools such as Meta and Google, to using custom solutions using proprietary MMMs such as those provided by XICA. There are many choices. Check the analysis and output functions of the model, consider the resources you can allocate to the project, and consider the method that is suitable for your company.
Whether you develop an MMM project in-house or collaborate with an external contractor, it is important to build a model that matches your business goals, report the analysis results to stakeholders, and proceed with the project while gaining their understanding. This is very important to achieve results through the project.
Additionally, when outsourcing to an MMM solution provider, it is important to consider analysis capabilities, customer support, price, track record, etc.
As MMM continues to receive renewed attention and refinement as a method for evaluating marketing effectiveness, understanding how to use MMM in practice has become an essential skill for brands and companies. I hope that the ``Practical Guide to Modern MMM (Marketing Mix Modeling) for Marketers'' that you have read this time will be of some help to you.
If you would like to learn more about XICA's data-driven marketing solutions, pleaseOur websitePlease refer to the. Also, if you are interested in how to implement an MMM project using XICA's services, please click here.Contact us.
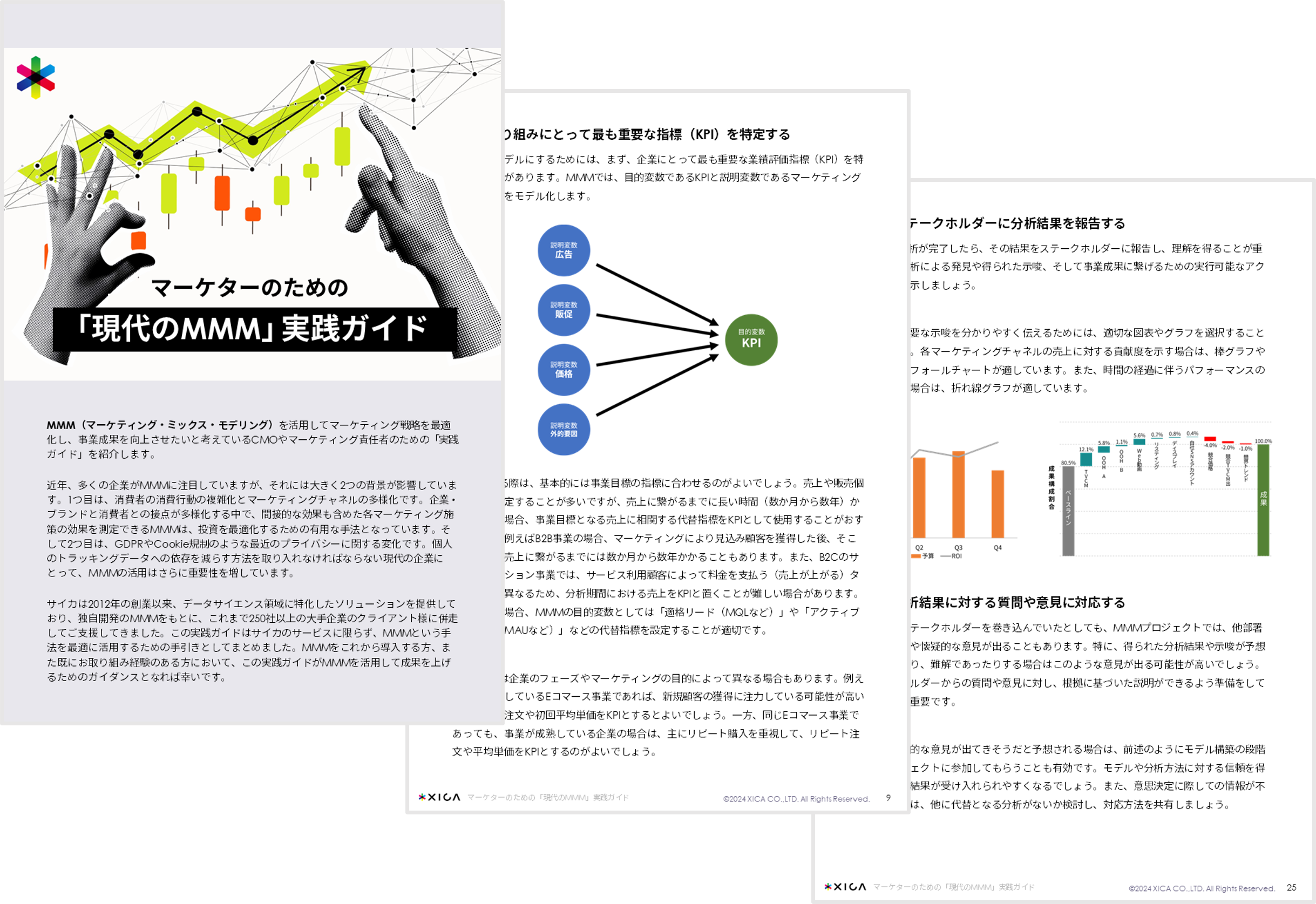
Click here for the material version of this article
A Practical Guide to Modern MMM for Marketers
~What are the key points to keep in mind to effectively utilize MMM, shared by XICA~
↓ Click here for a list of carefully selected articles to deepen your understanding of MMM
- What is MMM (Marketing Mix Modeling)? Explaining its features, procedures, examples, etc.
- Why you should use Marketing Mix Modeling (MMM) to measure the effectiveness of your advertising
- 10 Common Misconceptions About Marketing Mix Modeling (MMM)
- What are the limitations and areas in which MMM (Marketing Mix Modeling) does not excel?
- Latest Trends and Future Outlook of MMM (Marketing Mix Modeling) (2024 Edition))
- “Modern MMM (Marketing Mix Modeling)” Practical Guide for Marketers
Recommended articles
- Column
Where data and intuition intersect in marketing strategy: How to improve the quality and speed of your decisions
- Column
Turning environmental change into your ally: Marketing strategies to win in the market
- Column
The first step towards a data-driven marketing organization: A practical approach