【ゼロから始めるデータ分析#1】データ分析初心者がまず知るべき「分析の8ステップ」
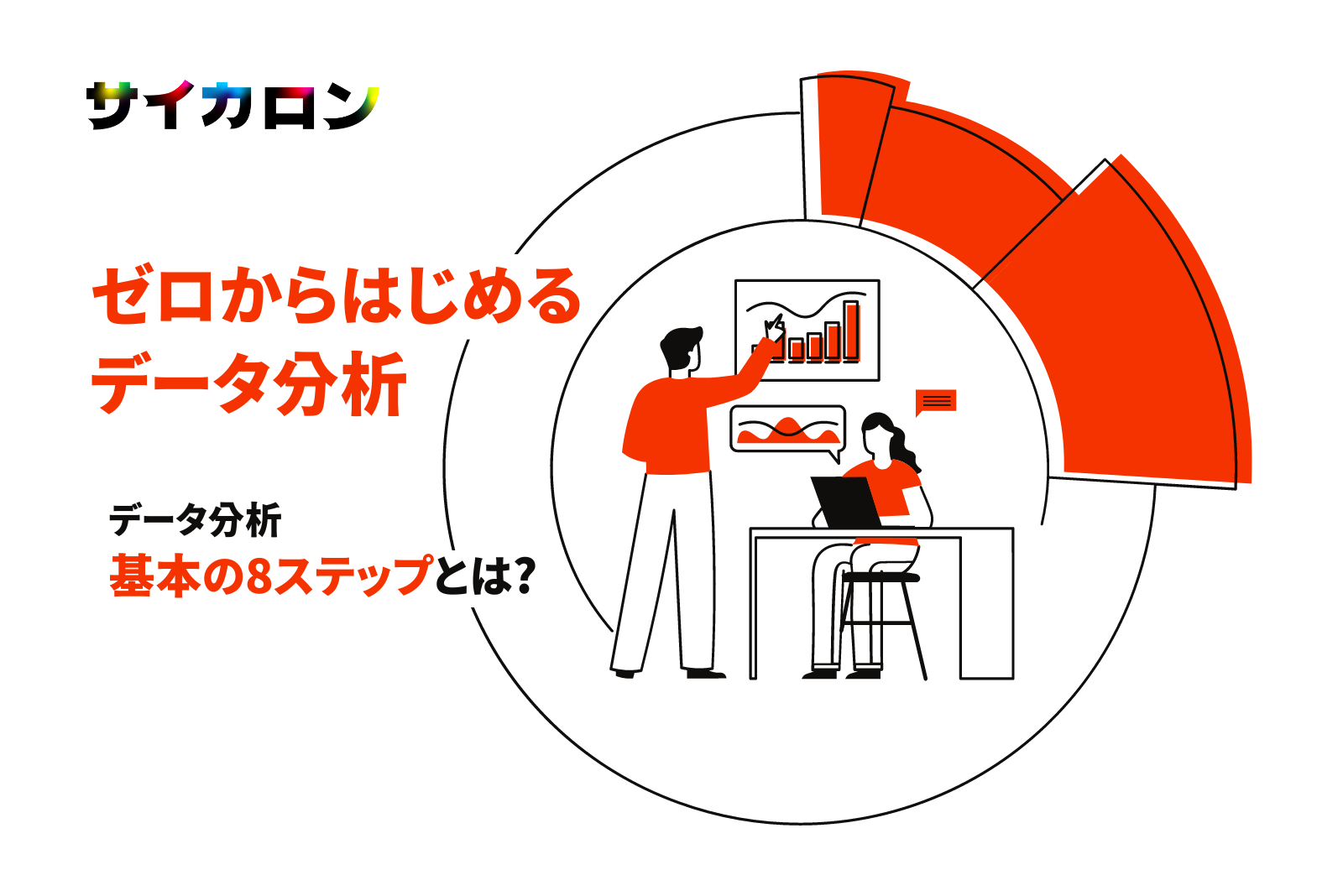
ビジネスにおいてデータ分析の重要性が増していることは周知の事実です。データドリブンな経営を志向し、すでに動き出している企業や組織も多いのではないでしょうか。
しかし、
- データ分析とは何なのか、実はよく分からない
- 貯まっているデータはあるが、目の前の課題との繋げ方が分からず活用できない
- データ活用を意識しているつもりだが、思ったような成果が出せていない
- 自分は文系で、統計学や数学、プログラミングに詳しくないからデータ分析はできない
と動き出せずにいるビジネスパーソンがいることも想像に難くありません。
ですが実際のところ、ビジネスでのデータ活用は、専門知識がなくてもできることが多いのです。
この連載では、データ分析を学んだことがない方に向けて、ビジネスにおけるデータ分析の必要性と覚えておくべきデータ分析の基本をポイントを絞って解説し、データをビジネスの成果に繋げるヒントを紹介していきます。
第1回となる今回は、データ分析の基本となる「データ分析基本の8ステップ」を解説します。
↓ 「ゼロから始めるデータ分析」記事一覧はこちら
#1 初学者がまず知るべき「分析の8ステップ」
#2 データ分析初心者が覚えておくべき3つの分析手法
#3 データ分析初心者が知っておきたい、経営層を巻き込むコミュニケーションのポイント
#4 データ分析初心者が知っておきたい、経営層がデータ分析と分析担当者に求めるもの
#5 データ分析初心者でも経営と組織を巻き込める、現場担当者のための4つのTips
目次
ビジネスにデータ分析が必要な理由
自動車を運転するときのことを考えてみてください。運転中、運転席前のメーターパネルに表示される速度計やガソリン残量、カーナビなどを確認しながら運転する方がほとんどではないでしょうか。
メーターがなくても車は走るし、目的地に到達することもできます。ですが、「メーターがない車を買いますか?」と聞かれたら、99.9パーセントの人はきっと買わないと答えるでしょう。
それはメーターが、「この速度でこのカーブを曲がり切れるか」「目的地までオイル補給なしで到達できるか」といったことを教えてくれるからです。また、カーナビが現在地や周辺情報を教えてくれるからこそ、いつも走ったことのない道を走る楽しみを味わうこともできるし、知らなかった抜け道を発見することもできます。
車のメーターは、目的地まで効率よく安全に行ける確度を高め、新しい道を教えてくれる、自動車にとって非常に重要な装置なのです。
データ分析を自動車のメーターに当てはめて考えると、なぜビジネスにデータが必要なのかがよく分かります。データを使わないビジネスは、メーターやカーナビのない自動車を運転しているようなものなのです。
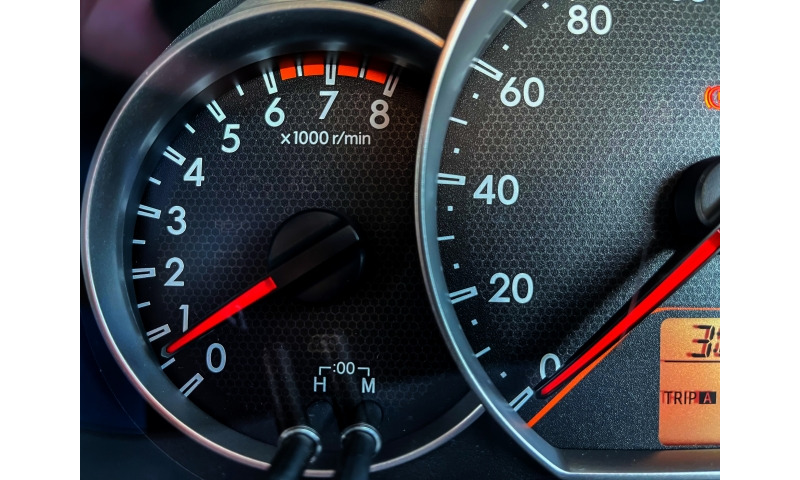
ビジネスにデータ分析を取り入れることで得られるメリットは、大きく2つあります。
一つは、「勝率を高めること」。
車のメーターが安全な速度や必要なオイル量を教えてくれるように、データを使うと勝率の高い戦略を導き出すことができます。成果に対して、どの要素がどのくらい影響を与えているのかを方程式化できるからです。成果と要素の関係性を可視化・数値化すると、成功に再現性がもたらされます。
データ分析を取り入れることで得られるもう一つの効果は、「これまで見落としていた欠点を浮き彫りにしたり、思いもよらなかった伸び代を顕在化させたりすること」です。意外にも、データはクリエイティブの源泉なのです。
データは、貯めれば貯めるほど強化されます。より学習できるようになり、精度が上がるからです。データの蓄積を始めるのが早ければ早いほど、ビジネスの勝率を高めることができます。
デジタル化がますます進む時代、これまで以上に“データに基づいた判断”が必要になってくるでしょう。
「データを活用する」とは
具体的なデータ活用のフローを解説する前に、「データ」「データ分析」「データ活用」がそれぞれ何を意味しているかについても整理しておきたいと思います。
① データとは
「データ」をあえて定義づけるなら、“世の中の事象を定量化したもの”といえるでしょう。つまり、どんなものでも定量化できればデータになるのです。
データとは何かと聞かれたとき、「2021年8月1日はのり弁当が20個売れた」という購買データや、「2021年7月の平均気温は25.9度であった」という気象データなどを想像する人が多いのではないでしょうか。
データは大きく「量的データ(量的変数)」と「質的データ(質的変数)」に分類できます。
量的データは、上に挙げたような個数や気温、件数、頻度、身長・体重など、単位のつく数値で表せるもの。一方の質的データは、性別や血液型、好きな芸能人や好き嫌いなど、カテゴリーを区別するものをいい、数値ではなく「あり・なし」や「A・B・O・A B」などの文字で表されます。

技術の進歩により、質的データでも定量化する工夫ができるようになってきました。これからは、データ分析で扱えるデータの種類がますます増えていくことが予想されます。
② データ分析とは
“データから情報を取り出すこと”をデータ分析といい、データ分析には「記述統計」と「推測統計」という2種類の手法があります。
記述統計は、集めたデータを図表やグラフにし、”データを見やすくして特徴を探る”分析の手法です。
たとえば、学年ごとの平均身長と平均体重を記録した数値データ。これらを棒グラフや折れ線グラフにすると、学年ごとの平均身長の差異や、身長と体重の関係性が見えやすくなります。このように、データを見やすく加工して、収集したデータの性質を把握する取り組みが記述統計です。
一方の推測統計は、一部のサンプル(統計学では「標本」という)から全体の傾向を捉え、”データを見てもわからない情報を取り出す”分析の手法です。
たとえば選挙速報。「開票率1%で当選確実」というニュースを見て、なぜ分かるのだろうと不思議に思ったことはないでしょうか。
統計分析はよく味噌汁に例えられます。鍋いっぱいに入っている味噌汁のうち、お玉ですくったひとすくいも同じ味噌汁です。選挙速報では、開票した表の一部をサンプルとして全体の傾向を探り、当選・落選を判断しています。このようにサンプルを取って全体を把握しようという取り組みが推測統計です。
全国で投票された選挙票をすべて開票するのが難しいように、すべてのデータを集めるのが困難なケースも多くあると思います。そのようなときにはこの推測統計を使います。
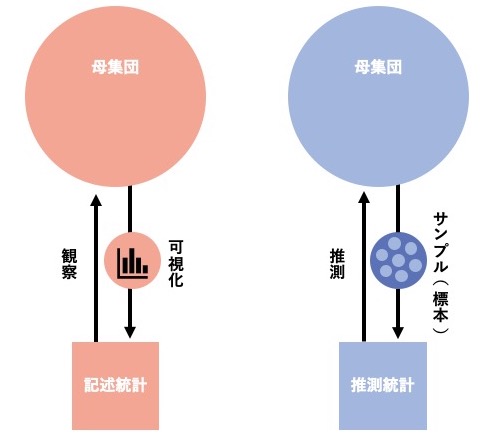
③ データ活用とは
データ分析によって、「身長と体重の増加は比例している」といった情報や、「男子は小学6年生から中学1年生の間の身長の伸びがもっとも大きい」など、何らかの情報が抽出されます。
“抽出された情報を目的に合わせて解釈し、適用する”のが「データ活用」です。
たとえば、「20:00以降にごはんを食べると太る」という分析結果(情報)があったとします。
この情報を、「スポーツのために体重を●●kg増やしたい」という目的を達成するために活用するのであれば、「夜ごはんの量をこれまでより●●パーセント増やそう」となります。けれどももし、「体重を適正体重まで落としてダイエットに成功したい」が目的であれば、「夜ごはんは●●時までに食べ終わっていたほうがよい」となるでしょう。
このように、目的が変われば分析結果の解釈とアクションは大きく変わります。ビジネスでデータを活用する際も、目的の設定は非常に重要です。
データ分析の8ステップ
ここからは、ビジネスにおけるデータ活用のフローを、8つのステップに分けて紹介していきます。
ビジネスでデータを活用するときにもっとも重要なのが、以下のフローに沿って進めることです。頭から順に進めていき、「おかしい」と思ったら前に戻る。このフローに従わずに進めると、そのデータ分析は失敗に終わる可能性が高くなってしまいます。
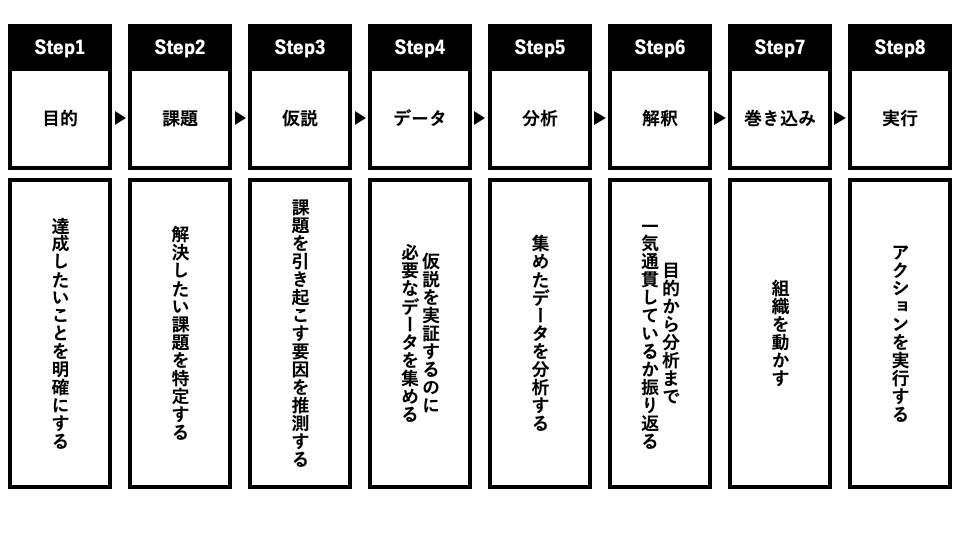
【Step1】 目的(達成したいことを明確にする)
先述のとおり、目的によって解釈やアクションは大きく変わります。なので、まずは“なぜデータ分析をするのか”という目的を明確にすることが重要。
「売上を最大化したい」「新規事業を成功させたい」など、会社としての大きな目標を自分ごと化し、データを活用して達成したい目的にまで落とし込む作業が、データ分析の最初のステップです。
Point)当たり前。でも重要な“目的意識”
一見当たり前に思われるかもしれませんが、目的があいまいなまま分析をした結果、多大な労力と費用をかけて分析をしたのに有益な示唆が得られずに終わるケースが多くあります。組織で分析・意思決定・巻き込み・実践への落とし込みを実現するためには、強い目的意識を持つことが重要です。
【Step2】 課題(解決したい課題を特定する)
データ分析の目的が明確になったら、目的を達成するために解決すべき課題を特定します。課題を特定するためのアプローチは2つあります。
1. 何が課題か想定できる場合:実データから特定する
例として、目的が「売上を最大化したい」の場合で考えてみましょう。
① 売上を構成する要素を分解する
売上は「新規売上」と「既存売上」に分解できます。さらにそれぞれの要素を分解していくと、売上を構成する要素の洗い出しができます。
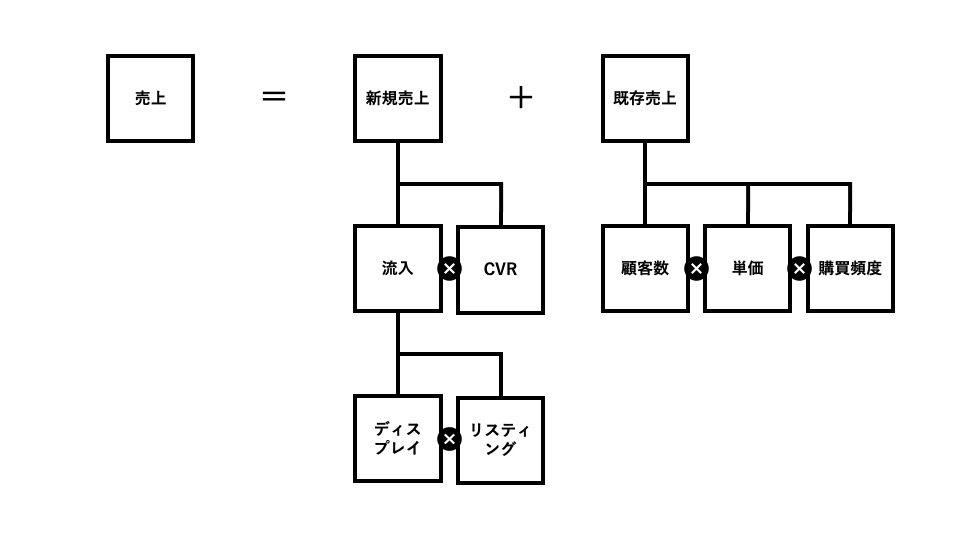
② 要素をシンプルなグラフにし、成果やコストを比較
要素の洗い出しができたら、上段から成果やコストを比較していきます。この時、シンプルなグラフにすると比較しやすいです。
売上を構成する「新規売上」と「既存売上」を比較すると、「既存売上」は安定している一方、「新規売上」が下がっていることがわかります。
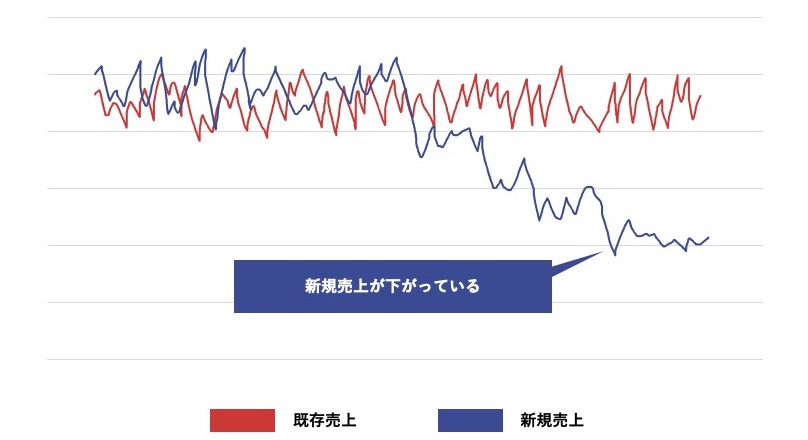
「新規売上」が下がっていることがわかったので、次に「新規売上」を構成する「CVR」と「流入」を比較します。「CVR」は安定している一方、「流入」が低下していることがわかります。
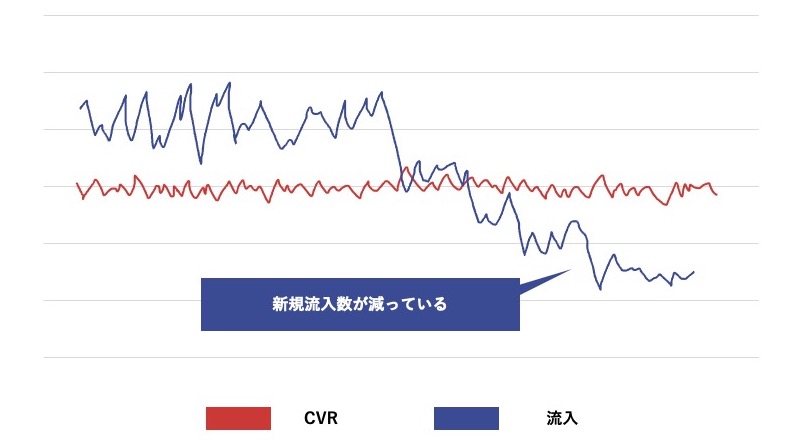
③ 課題を特定
同じように、低下している「流入」を構成する「ディスプレイ広告(からの流入)」と「リスティング広告(からの流入)」をグラフで比較してみると、リスティング広告からの流入数が低下していることがわかります。
ここで、リスティング広告の流入数低下が課題であると特定できます。
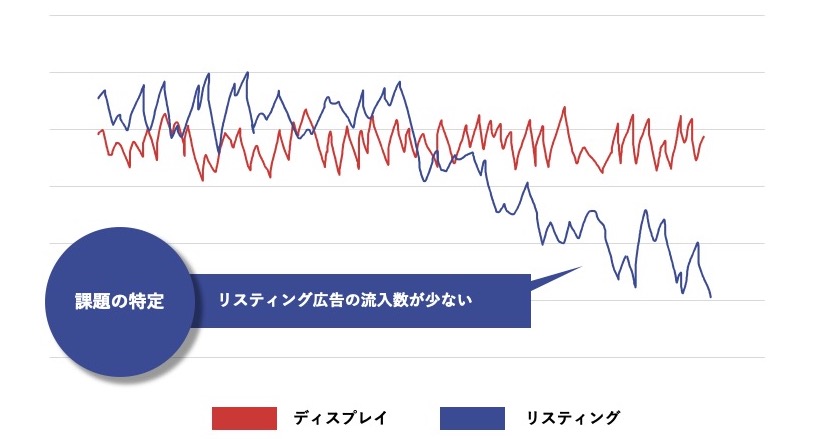
2. 過去のデータがなく、何が課題か想定できない場合(新規事業を創出する場合など):未来の仮説(こうなるのではないか)をつくり、想定される未来の課題を洗い出す
① 未来の仮説を立てる
② 仮説に近い過去のデータを参考に、因果関係を推測する
③ 課題を特定する
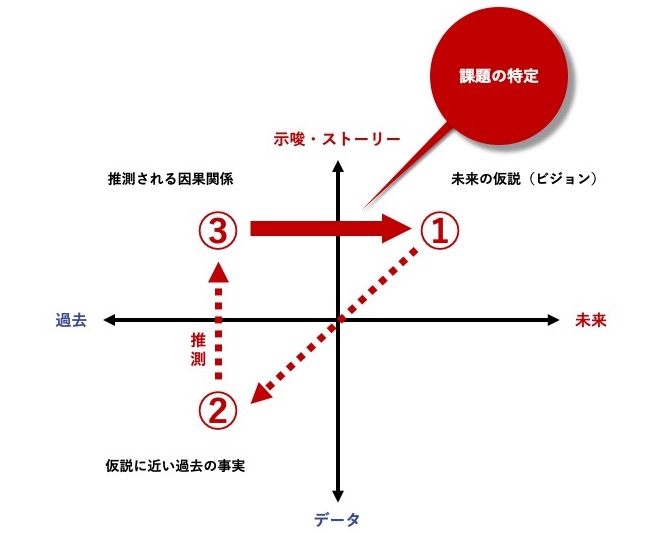
Point)課題は、2つのアプローチのいずれかを使って特定する
これらのアプローチを使わずに課題を特定しようとすると、妄想で課題を設定することになります。そうすると、課題を達成しても目的が達成されないという落とし穴にハマってしまうので注意が必要です。
【Step3】 仮説(課題を引き起こす要因を推測する)
課題が明確になったら、Step3で、その課題を引き起こしている要因を推測します。「Step2:課題の特定」と同じように、ここでも要素の洗い出しと構造化からスタートします。
例として、「リスティング広告の流入数が少ない」という課題の要因を推測してみましょう。
① 「リスティング広告の流入数」を構成する要素を洗い出し、洗い出した要素を構造化する
課題と特定した「リスティング広告の流入数」を構成する要素を洗い出し、課題→KPI→要因の順番で構造化していきます。
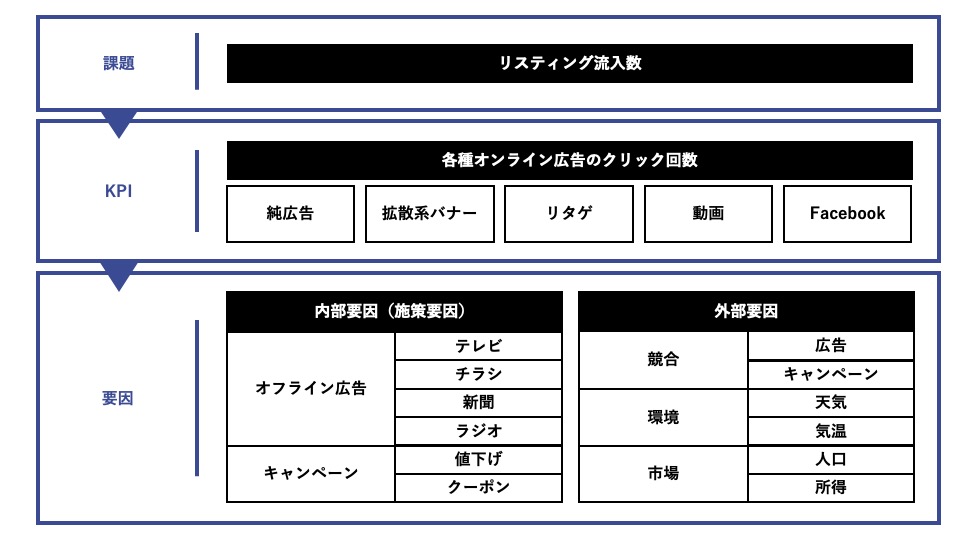
Point)構造化したら、「因果関係は正しいか」「MECEになっているか」の2点を確認する
構造化する中で、因果関係の間違いや要素の抜け漏れ・重複があると、精度の高い仮説が立てられません。構造化したら、以下の4点を確認しましょう。
・ KPIと要因(施策)が同じステップで扱われていないか
・ 課題とKPI/KPIと要因が逆になっていないか
・ 課題を説明する要素に漏れがないか
・ 要素に重複はないか
② 課題を引き起こす要因を推測する
構造化した図を見ながら、どの要因が課題を引き起こしているのかを推測します。
Point)仮説を立てる際は、チームメンバーや組織外の人と意見交換をする
自身の経験が仮説の範囲を狭めてしまったり、仮説の矛盾に気づかないまま進めてしまったりするケースがよくあります。仮説を立てる際は、社内外の人の意見を聞き、仮説の精度を高めることが重要です。
③ 想定される分析結果を推測する
②で課題を引き起こす要因を推測したら、それらの要因がどのくらい影響を及ぼしているのかまで推測するようにしましょう。
Point)仮説をつくる時点で、想定される分析結果も想定しておく
仮説を立てたら、“その仮説を実証したらどのような分析結果が出るか”まで考えておきましょう。
たとえば、「売上低下には、値下げと天気が影響している」という仮説を立てたときは、「値下げは売上の○○パーセント、天気は○○パーセント程度に影響を与えている」といった分析結果まで想定するようにします。
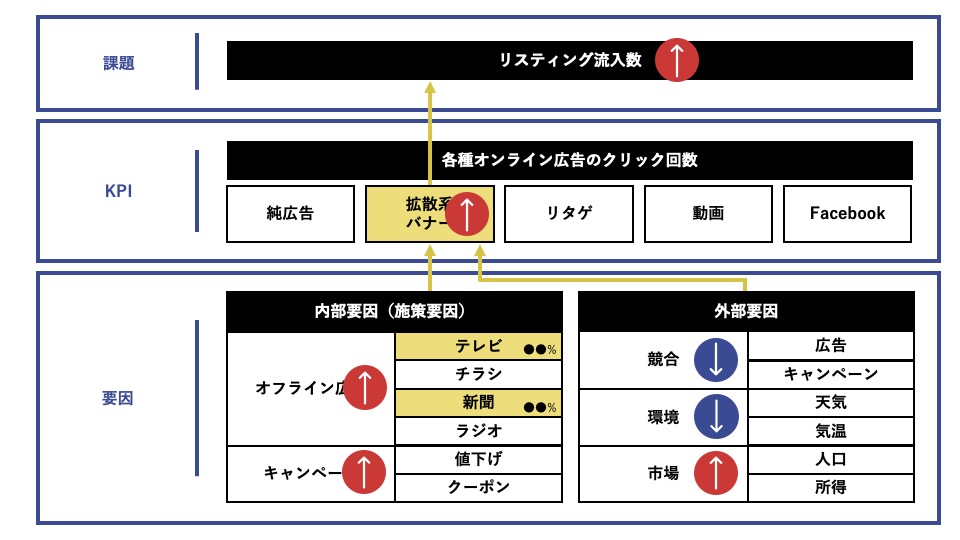
【Step4】 データ(仮説を実証するために必要なデータを集める)
「Step4:データ」では、Step3で立てた仮説を実証するために必要なデータを集めます。
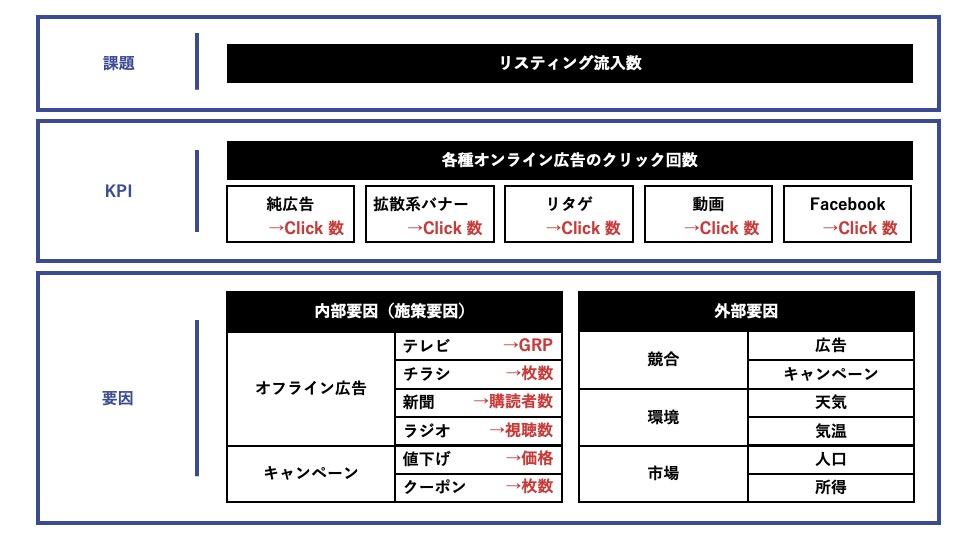
Point)データを集める際は、“仮説を実証するために必要なデータは何か”という視点で考える
持っているデータをそのまま使って仮説を実証しようとすると、場合によっては間違った分析をしてしまいます。以下の例を参考に、“仮説を実証するために必要な形”を考えましょう。
(例)持っているデータをそのまま使わず、“仮説を実証するために必要な形に変えて”使う例
「夏の暑い日ほど売上が落ちる」という仮説を実証したい場合:
「8/1は30度、8/2は32度……」といった気温データをそのまま使って分析をする
→「季節を問わず、気温が1度上がると売上が●●円下がる/上がる」という、夏に限らない分析結果が得られ、仮説を実証できない。場合によっては、誤った分析の示唆を導き出してしまう。
「気温30度以上の日は1、30度未満の日は0」といったフラグを立てて分析をする
→「30度以上の日に売上が●●円下がる/上がる」のような、気温と売上の関係性が分かる分析結果が得られ、仮説を実証できる。
【Step5】 分析(集めたデータを分析する)
集めたデータを、仮説を証明するために適切な分析手法で分析します。
(※詳細は、第2回の記事で解説します)
【Step6】 解釈(目的から分析までが一気通貫しているか振り返る)
解釈のステップは、このあとアクションを実行すべきかを判断する最後のとりで。目的から分析までが一気通貫しているか否かを振り返る作業=解釈、と考えると分かりやすいです。
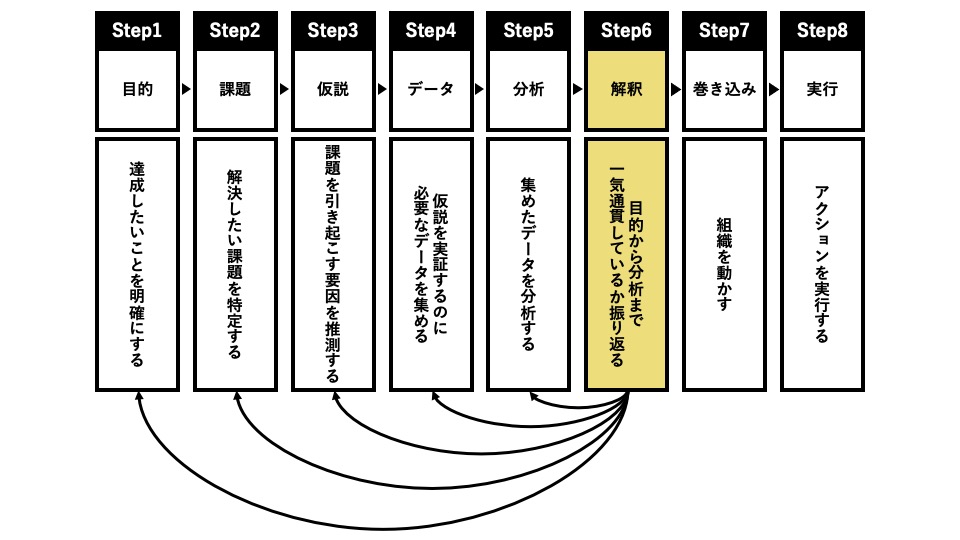
Point)振り返りは、Step3で立てた“仮説” と “想定した分析結果” を、実際の分析結果と見比べて行う
(一フェーズずつ戻って確認する必要はありません)
1. 仮説も分析結果も、当初想定していたものと違う場合
① 「Step1:目的」に戻り、“どういう目的で分析をしたのか” “特定した課題は何だったか”を確認する
② 最初に立てた仮説とは別の因果関係をもとに仮説を立て直す
③ 再度、「Step4:データ」「Step5:分析」「Step6:解釈」と進める
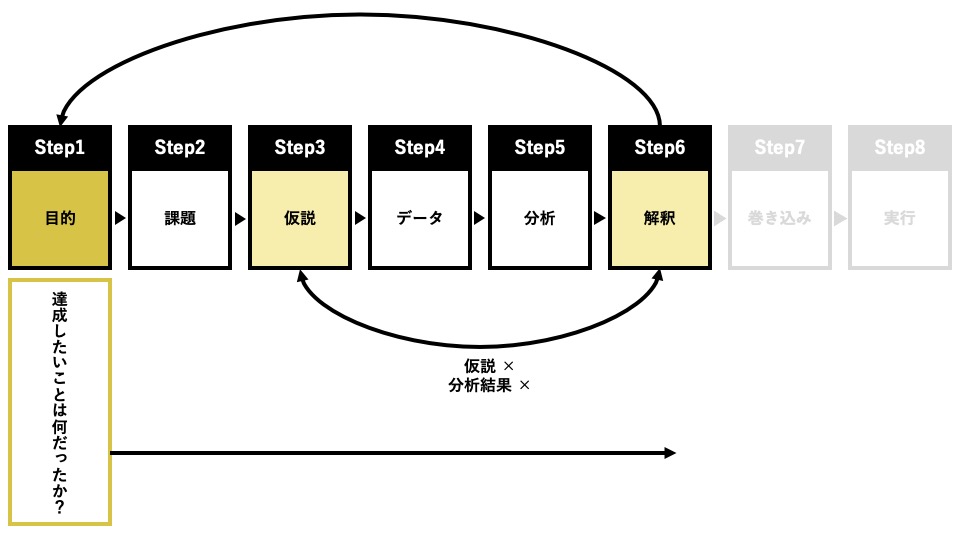
2. 仮説は合っているが、分析結果が当初の想定と違う場合
① 「Step4:データ」に戻り、データに間違いがないかを確認する
② 正しいデータを集める
③ 再度「Step5:分析」「Step6:解釈」と進める
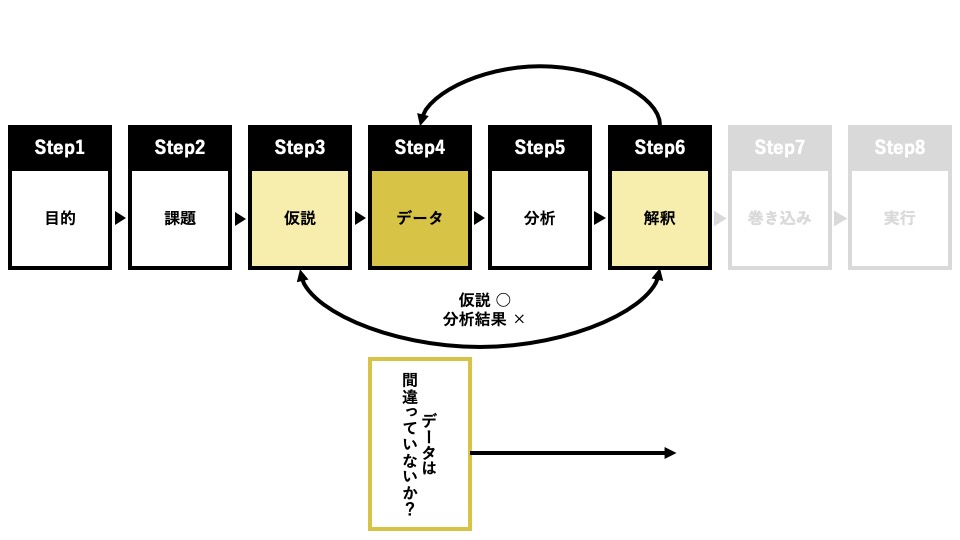
【Step7】 巻き込み(データをもとに組織を動かす)
仮説が実証されたら、組織を巻き込んでアクションの実行に進みます。
(※詳細は、第3回、第4回の記事で解説します)
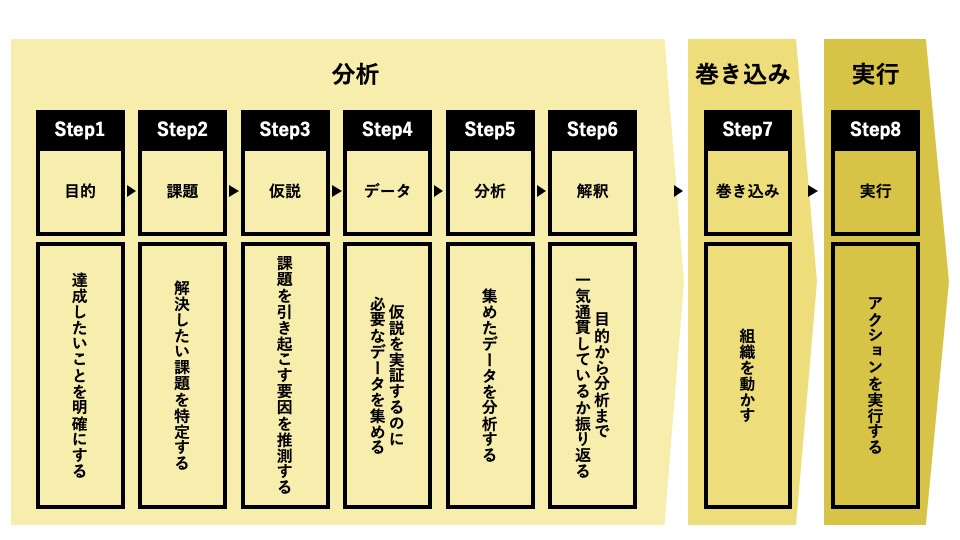
【Step8】 実行(決定したアクションを実行する)
データ分析をゼロから学びたい人におすすめの書籍3選
西内啓(2013)『統計学が最強の学問である』ダイヤモンド社
統計学は何のためにあるのか。何の役に立つのか。現代社会と我々の人生に、統計学が与えるインパクトの大きさを実感できる一冊です。
鳥居泰彦(1994)『はじめての統計学』日本経済新聞出版
数学が苦手な人でも理解できるよう、丁寧に解説された統計学の入門書。統計学とは何かを体系的に理解するためにまず読みたい一冊です。
森岡 毅・今西 聖貴(2016)『確率思考の戦略論』KADOKAWA/角川書店
ユニバーサル・スタジオ・ジャパン(USJ)のV字回復の裏に存在した「数学マーケティング」について詳細に書かれています。データ活用の具体的な事例がイメージできる一冊です。
※Amazon商品ページのリンクを掲載しています
おわりに
以上、データ分析の8ステップを紹介してきました。
この8ステップを順番にやらないと、目的不在のデータ分析になりやすく、データ分析をアクションの実行まで繋げられない可能性が高まります。
特に、「こんな分析がしたい→こんなデータがほしい→こんな仮説もあるのでは?」と、フローを逆走していくパターンは、いちばんよくないデータ分析だといえます。アクションに繋げられないデータ分析はビジネスで活用できず、分析のための分析で終わってしまいます。
この8ステップを見てもらうと分かるとおり、数学や統計分析の専門知識を必要とするのは「Step5:分析」だけ。データ分析で重要なのは、明確な目的意識と精度の高い仮説、経験が育てる想像力と創造性です。すでに業務に関わっている方なら、これらはきっと持ち合わせていると思います。
はじめてデータ分析をする方も、ぜひ一度、この8ステップを一気通貫で体感してみてください。
↓ 「ゼロから始めるデータ分析」記事一覧はこちら
#1 初学者がまず知るべき「分析の8ステップ」
#2 データ分析初心者が覚えておくべき3つの分析手法
#3 データ分析初心者が知っておきたい、経営層を巻き込むコミュニケーションのポイント
#4 データ分析初心者が知っておきたい、経営層がデータ分析と分析担当者に求めるもの
#5 データ分析初心者でも経営と組織を巻き込める、現場担当者のための4つのTips
↓ ビジネスメディア『PIVOT』にて、ビジネスにデータサイエンスを活かす方法を解説しています
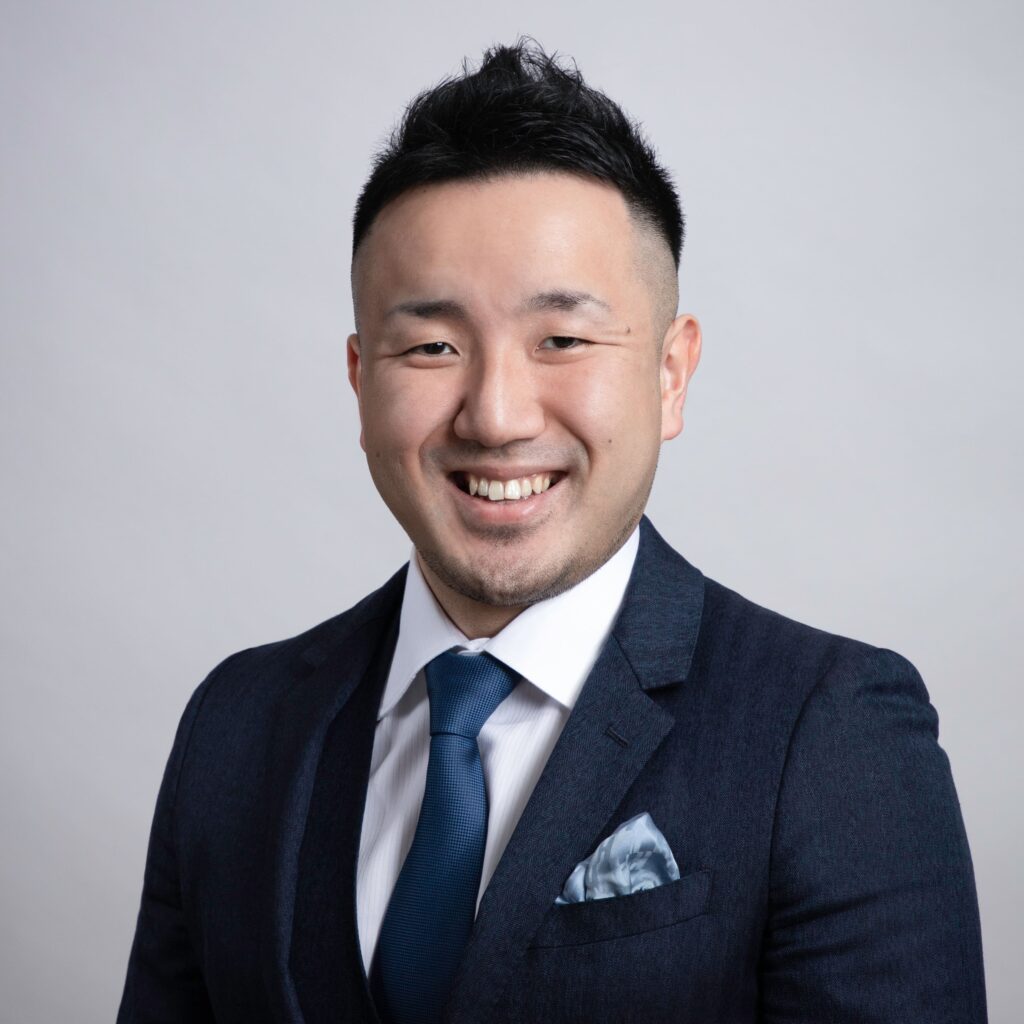
株式会社サイカ
代表取締役CEO 平尾 喜昭
父親の倒産体験から「世の中にあるどうしようもない悲しみをなくしたい」と強く思うようになる。慶應義塾大学総合政策学部在学中に統計分析と出会い、卒業直前の2012年2月、株式会社サイカを創業。創業前にはバンドマンであったというユニークなキャリアも持つ。