Data science as a means of leveraging “experience and intuition”
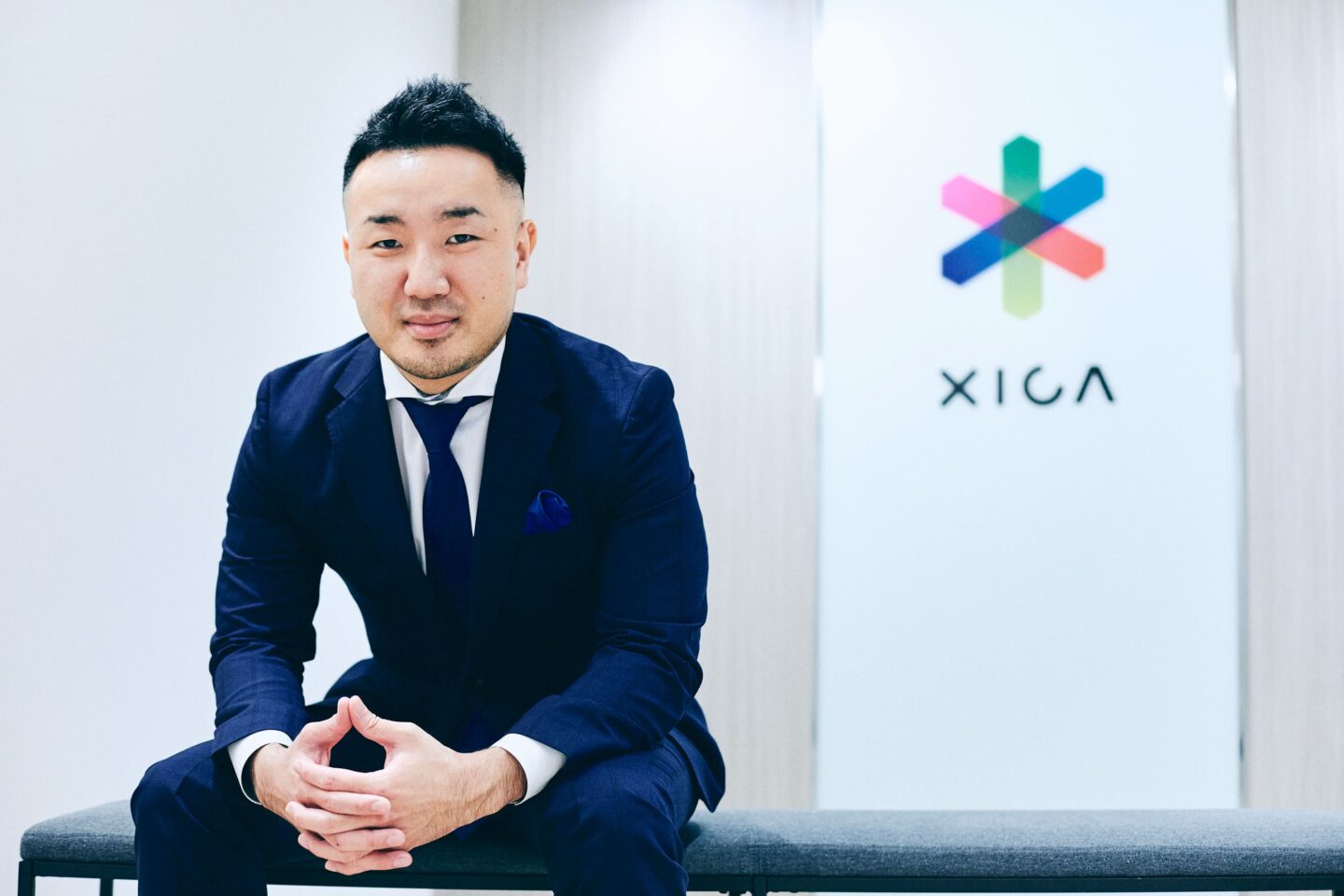
Many successful companies, such as Amazon, Walmart, and Netflix, are actively using data, and the importance of data in achieving business results is widely known. Even so, many people are still uncomfortable with data, and feel out of touch with it.
In this article, we take a fresh look at what can be achieved through data science and its enormous potential, and ask Yoshiaki Hirao, the CEO of XICA, about the meaning and significance of data science for companies today.
XICA CEO Yoshiaki Hirao
A graduate of Keio University, Faculty of Policy Management. Yoshiaki witnessed the bankruptcy of his father’s company, and this drove him to discover the potential of management support through statistical analysis in university. After spending some time as a musician, he founded XICA Co., Ltd. in February 2012.
Contents
- Expectations for data science to achieve “discontinuous growth”
- The two effects of data science: “increased certainty” and “unexpected discoveries”
- The most important factors in data science: Experience and intuition
- Data science bringing us into an era where we can compete on the basis of “human abilities”
Expectations for data science to achieve “discontinuous growth”
– What do you see as the reason for the rapid increase in attention to data science in recent years?
Compared to 2012, when XICA was founded, there has been a drastic change in the situation surrounding data science. Although data science was considered important back then, it is only in the last five years or so that it has come to attract attention from a wider audience.
The dawn of data science took place when the importance of collecting and storing data started being widely discussed with the trend of “big data”. Interest in processing and organizing the collected data grew. Around this time, the market grew significantly, as companies offering BI (Business Intelligence: organizing and visualizing big data to support decision-making in management) tools went public one after another.
In recent years, the need to analyze and leverage the data for future actions has increased, and naturally, data science has come to the forefront of attention.
It is meaningless to simply “collect,” “accumulate,” and “organize” data; it is necessary to “analyze” it in order to achieve goals and solve issues – a conclusion that seems obvious in retrospect. However, as the amount of data circulating in the world has become vast and diverse, and tools for managing and utilizing data have become widely available, the number of people and companies who want to make effective use of data has gradually increased over the past decade.
In addition to this, several events spurred the growing momentum for data utilization.
The most significant of these events that turned the tide was the publication in 2013 of “Statistics: literacy for the next generation” by Hiromu Nishiuchi. Statistics and data science became widely known and recognized by the general public, including those who were not familiar with data. I believe it was during this period that data scientists and other people who handle data began to be recognized as “cool (and able to earn a good living)”.
It was around the same time that the movie “Moneyball” (*1) was released, and GAFA grew at an accelerated pace. Stories of success about people and companies by making use of data were widely showcased on various media, and data science rapidly became an object of attention.
One thing to note is that every one of these stories of success was an episode of “discontinuous growth” through the use of data.
By leveraging data, the Oakland Athletics team in “Moneyball” made the playoffs four years in a row starting in 2000, twice winning more than 100 games in a season during that time period. Initially small startups, GAFA has risen discontinuously to become major players in the industry by leveraging data to its advantage.
I believe that data revealed things that had not been seen or noticed before, and people were further attracted to data science by the expectation that they could win even with limited resources if they made full use of data.
In an age where it has become difficult to succeed or survive by simply continuing to do the same thing as the past, I believe that data science has been embraced as a tool for success that many people and companies have been waiting for.
*1: “Moneyball” is a film about the life of Billy Beane, the real-life general manager of a Major League Baseball team, who transformed a substandard team into an all-time winning team through his unique theory, the Moneyball Theory. The Moneyball Theory uses “sabermetrics,” which objectively evaluates players based on various statistics.
The two effects of data science: “increased certainty” and “unexpected discoveries”
– What is it exactly that data science can achieve?
In a nutshell, it is to “increase certainty”. Whether in politics, business, or sports, the power of data science is that it enables us to increase the probability of a success, and lock in a win.
So data science can be described as “the process of deriving the laws of success”.
There are generally two ways to “increase certainty” using data. One is to prove a hypothesis, and the other is to gather clues to formulate a hypothesis.
Take, for example, a company’s advertising activities. Data can be used to test the hypothesis “In order to increase sales, shouldn’t we strengthen ad B rather than ad A?” If the result is contrary to the hypothesis, the possibility of new advertising media and creative output can be explored.
Of course, advertising is not the only area where data science can be utilized. The following are just a few examples of what has been achieved through data science.
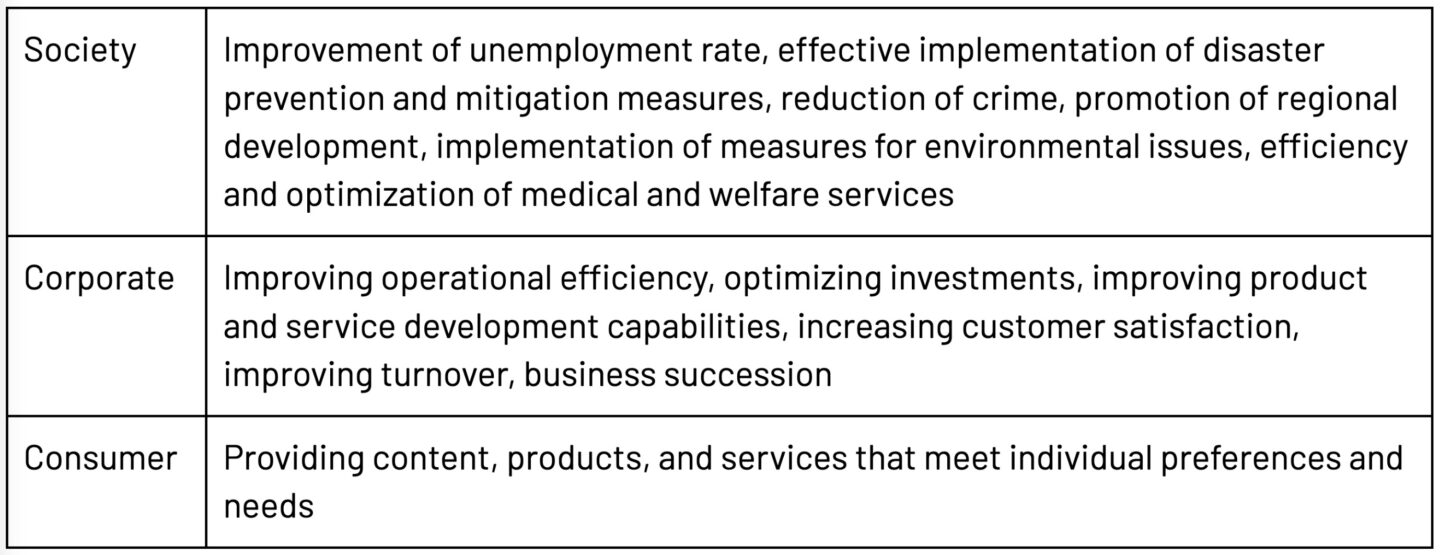
As long as we can convert events into data, we can utilize data science.
Thanks to advances in technology, almost everything in the world can be converted into data in some form, and any data can be analyzed in some way. It is no exaggeration to say that there is no objective or problem that cannot be achieved or solved by data science.
Because it can be used to achieve any goal or solve any problem, there are countless examples of companies that have succeeded through the use of data, regardless of the size of the company or type of business.
Netflix started its DVD software rental and distribution business by mail in 1998 with only 30 employees. The company hired a data scientist with programming expertise and used data analysis to drive recommendation functions, personalization, and the creation of original productions. This has led to exponential growth, with Netflix achieving sales of $25 billion (approximately 2,591.5 billion yen) in 2020 (*2).
Walmart has risen to become the world’s largest retail chain with the power of data analytics. The company was adept at analyzing not only quantitative sales and inventory data, but also qualitative data, such as region-specific needs and changes in needs due to weather. The company is also known for providing the resulting data and analysis to its suppliers, thereby driving growth in the industry as a whole (*3).
Making full use of data to improve business results is becoming a standard in any business, so much so that we probably didn’t even have to convince you by bringing up these examples.
*2:Sources: “Competing on Analytics (Thomas H. Davenport and Jane G. Harris, Nikkei BP, 2008),” “Harvard Business Review (https://hbr.org/2018/01/data-can-enhance-creative- projects-just-look-at-netflix),” “Netflix, Inc. Financial Results (https://s22.q4cdn.com/959853165/files/doc_financials/2020/q2/FINAL-Q2-20- Shareholder-Letter-V3-with-Tables.pdf)”
*3:Source: “Competing on Analytics (Thomas H. Davenport and Jane G. Harris, Nikkei BP, 2008)”
The most important factors in data science: Experience and intuition
– How exactly does data science proceed?
Data science consists of eight basic steps.
1. Objective: What is your goal?
2. Problem: What is the issue?
3. Hypothesis: What are the factors causing the issue?
4. Data: What is the necessary data?
5. Analysis: What kind of analysis should be run?
6. Interpretation: How should the data be interpreted?
7. Involvement: How to take the next steps as an organization
8. Execution
In this overview of the 8 steps, the word “data” appears right in the middle of the entire process. This means that even if you start doing “data science,” that does not necessarily mean that you will suddenly start by dealing with data. In addition, expertise in statistics and data analysis is only required in the 4th and 5th steps.
In fact, there are many more elements to “data science” than “data” and “analysis”. Many people think of data science as something that only people in the sciences do, or something that requires expertise in statistics, but this is a major misconception.
Rather, the most important thing in data science is to set a direction for data analysis with a “objective,” “problem,” and “hypothesis”. Then, in the interpretation process, we must find relationships between the data presented before us and create a story.
If this is neglected, no matter how much data is obtained, and how accurate the analysis methods are, it will not be possible to obtain effective suggestions from the data or to link the analysis results to business results.
In other words, as long as the “objectives,” “issues,” “hypotheses,” and “interpretations” are in place, the “data” and “analysis” can be left to the experts.
– So data science can be practiced even by people who are more involved in liberal arts rather than tech or the sciences?
In fact, there may be many cases where “liberal arts” people who have a vague sense of aversion to data science may be more suited to data science.
It is important to imagine causal relationships among various phenomena, and to formulate hypotheses. This “hypothetical ability” is an indispensable skill for practicing data science.
There is a story about a convenience store chain that once hired a large number of data scientists to analyze data in order to find “factors that cause sales,” and the answer they derived was quite obvious: “Sales go down when it rains.” Of course, this result in itself is not wrong, but it is an anecdote that clearly shows that it is impossible to obtain valid suggestions from data by analyzing them without a hypothesis.
Without a hypothesis, some events would not be converted to data, and would not be analyzed. In other words, there is information that cannot be noticed or obtained without the ability to hypothesize. As analytical technologies become commoditized, “the ability to hypothesize” will give rise to superiority not only in data analysis, but also in business itself.
We believe that the foundation of this ability to hypothesize is the “experience and intuition” of each individual.
For example, rain is said to have a negative impact on store sales. When analyzing data on this matter, if a hypothesis such as “sales go down when it rains” is explored in depth as shown below, the possibility of obtaining valid suggestions will increase.
- Examine the amount of rainfall based on the hypothesis that the more it rains, the lower sales will be.
- Based on the hypothesis that sales will decrease when the amount of rainfall exceeds a certain level, examine the relationship between the amount of rainfall and sales.
- Based on the hypothesis that sales differ depending on the amount of precipitation, we categorize precipitation into ○ mm to ○ mm / △ mm to △ mm / □ mm to □ mm, and examine the impact of each on sales.
- Under the hypothesis that the combination of precipitation and temperature affects sales, the relationship between precipitation as well as temperature and sales will be examined.
The experience of retailers will be a great advantage in formulating these hypotheses and analytical plans. Having the experience that “a certain number of customers come to the store even on rainy days,” or the intuition that “sales seem to drop on days when it rains and the temperature is high,” allows one to formulate a more accurate hypothesis, and think of appropriate ways to convert it into data.
It can said that data science is a tool for maximizing the “experience and intuition” of each individual, including managers and marketers, in achieving goals and solving problems.
Data science bringing us into an era where we can compete on the basis of “human abilities”
– It seems surprising that “experience and intuition” can be assets in data science.
There was a time when it was said, mainly in the marketing industry, that “data science is a method of discovering laws and improving reproducibility based on data, rather than relying on human experience and intuition,” but this is a big mistake.
This erroneous perception has created resistance to data science among general businesspersons other than data scientists, and it has been one of the factors that have hindered the spread of data science.
Data science is a means to maximize human experience and intuition. By spreading this correct understanding, I hope more people and companies will practice data science.
– How will society change when more people and companies start practicing data science?
I believe that society will become a place where people can say, “I am fully prepared, all I need to do now is to compete with my own talent.” In fact, it was my desire to create such a society that led me to establish XICA.
Currently, there are “haves and have-nots” in data science. In other words, there is a polarization between companies that are practicing data science and those that are not, and the former continue to win.
If we can spread data science to more people and companies, we will be able to compete on the basis of “human abilities.” In business, I believe that the time will come when we will be able to make better use of our uniquely human abilities, such as someone’s understanding of customer perspective cultivated through continuous interaction with customers, or an eye for identifying trends in society and the market, our unique sensibilities and feelings, and the courage and passion to take the time to face things.
I hope to create a world where people can compete with their own talents without the frustration of thinking, “If only I had more resources (people, goods, money, and information), I could have won.” That is my goal and the potential of data science.
Interviewed and written by Chiaki Saito
Photographed by Daisuke Koike
Planned and edited by Yuko Kawabata (XICA)